Ap Statistics Unit 7 Progress Check Mcq Part C
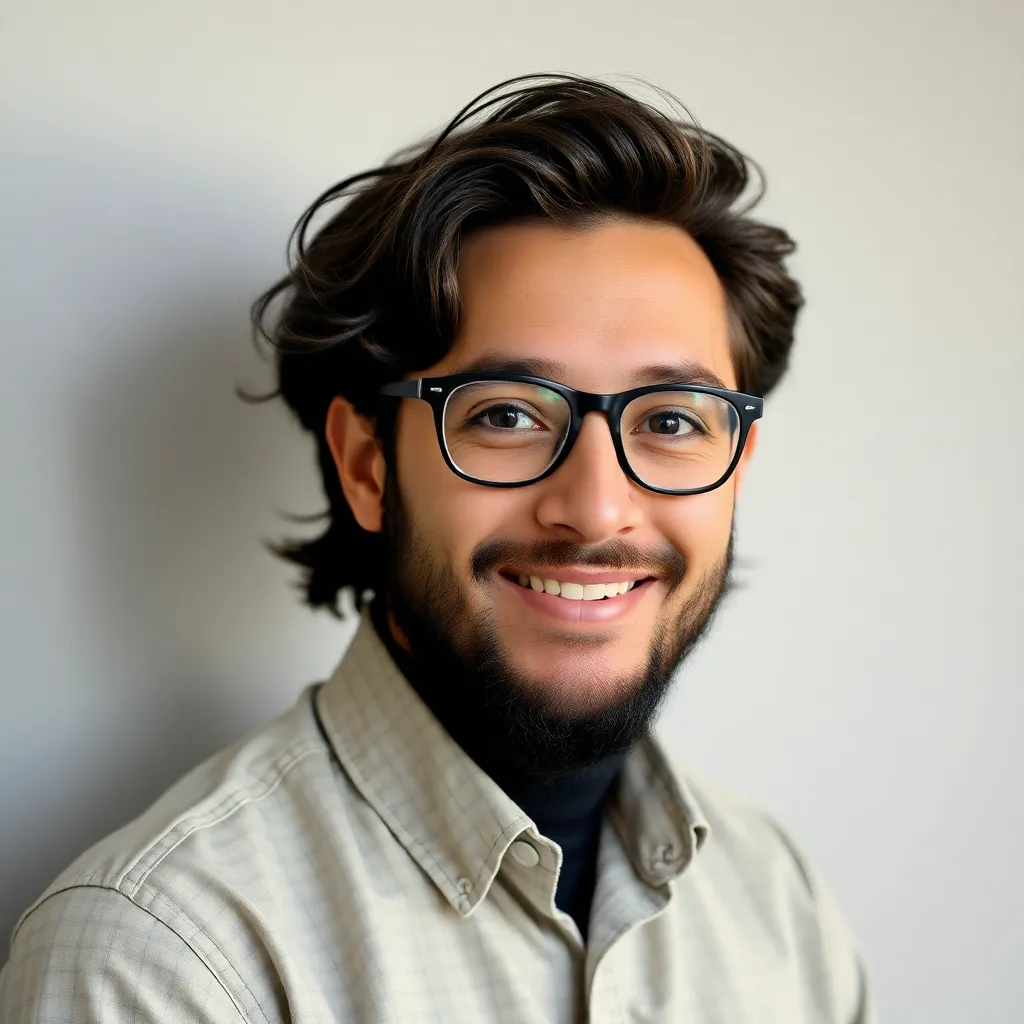
New Snow
Apr 22, 2025 · 7 min read

Table of Contents
AP Statistics Unit 7 Progress Check: MCQ Part C – A Deep Dive
Unit 7 of AP Statistics, focusing on sampling distributions, is notoriously challenging. The Progress Check MCQs, particularly Part C, often present complex scenarios requiring a strong understanding of concepts like sampling variability, central limit theorem, and confidence intervals. This comprehensive guide will dissect the key concepts within Unit 7 and provide strategies for tackling the challenging MCQ Part C questions.
Understanding the Fundamentals: A Review of Unit 7 Concepts
Before diving into specific problem types, let's solidify our understanding of the core concepts tested in Unit 7:
1. Sampling Distributions
The heart of Unit 7 lies in understanding sampling distributions. A sampling distribution isn't a distribution of the data itself, but rather a distribution of statistics calculated from many different samples drawn from the same population. For example, if we repeatedly take samples of size 50 from a population and calculate the mean of each sample, the distribution of these sample means forms the sampling distribution of the mean.
Key Characteristics:
- Center: The center of a sampling distribution of the mean is typically close to the population mean (μ).
- Spread: The spread (standard deviation) of the sampling distribution is smaller than the population standard deviation (σ). It's given by σ/√n, where n is the sample size. This is crucial for understanding the impact of sample size.
- Shape: The Central Limit Theorem (CLT) states that for large enough sample sizes (generally n ≥ 30), the sampling distribution of the mean will be approximately normal, regardless of the shape of the original population distribution. This is a cornerstone of inferential statistics.
2. The Central Limit Theorem (CLT)
The CLT is arguably the most important theorem in Unit 7. It allows us to make inferences about a population even if we don't know its distribution. As long as the sample size is sufficiently large, the sampling distribution of the mean will be approximately normal. This normality allows us to use familiar tools like z-scores and the normal distribution to calculate probabilities and construct confidence intervals.
Implications of CLT:
- Normal Approximation: We can use the normal distribution to model the sampling distribution of the mean, enabling us to calculate probabilities associated with sample means.
- Inference: The CLT forms the foundation for many statistical inference procedures, including hypothesis testing and confidence interval construction.
3. Confidence Intervals
Confidence intervals provide a range of plausible values for a population parameter (like the mean or proportion). They are constructed using sample statistics and a margin of error, which accounts for sampling variability. A 95% confidence interval, for instance, means that if we were to repeat the sampling process many times, 95% of the resulting confidence intervals would contain the true population parameter.
Key Components of a Confidence Interval:
- Point Estimate: The sample statistic (e.g., sample mean) used as the center of the interval.
- Margin of Error: The amount added and subtracted from the point estimate to create the interval. This margin accounts for the uncertainty due to sampling variability. It's influenced by the sample size (larger samples lead to smaller margins of error) and the confidence level (higher confidence levels lead to larger margins of error).
- Confidence Level: The probability that the interval contains the true population parameter.
Tackling AP Statistics Unit 7 Progress Check MCQ Part C: Strategies and Examples
Part C questions typically require a deeper understanding and application of these concepts. They often involve:
- Interpreting sampling distributions: Questions might ask you to interpret the shape, center, and spread of a sampling distribution given a scenario.
- Applying the CLT: You might need to determine whether the CLT applies in a given situation and use it to approximate probabilities or construct confidence intervals.
- Understanding the impact of sample size: Questions often assess your understanding of how sample size affects the precision of estimates and the width of confidence intervals.
- Combining concepts: Part C questions frequently combine multiple concepts, requiring you to synthesize your knowledge to arrive at the correct answer.
Let's illustrate with examples:
Example 1: Interpreting a Sampling Distribution
A researcher is studying the average height of adult women in a certain city. She takes many random samples of size 50 and calculates the mean height for each sample. The resulting distribution of sample means is approximately normal with a mean of 64 inches and a standard deviation of 0.8 inches. Which of the following statements is NOT true?
(a) The sample size is large enough for the Central Limit Theorem to apply. (b) The mean height of adult women in the city is approximately 64 inches. (c) The standard deviation of the heights of all adult women in the city is 0.8 inches. (d) A sample mean of 66 inches would be considered unusually high.
Solution:
(a) is true because n=50 is generally considered large enough for the CLT. (b) is likely true. The mean of the sampling distribution is a good estimate of the population mean. (c) is FALSE. The standard deviation of 0.8 inches refers to the standard deviation of the sampling distribution, not the population. The population standard deviation would be larger. (d) is true. A mean of 66 inches is more than 2.5 standard deviations away from the mean of the sampling distribution, making it unusual.
Example 2: Applying the CLT and Confidence Intervals
A company wants to estimate the average lifespan of its light bulbs. They randomly sample 100 light bulbs and find that the average lifespan is 1000 hours with a standard deviation of 100 hours. Construct a 95% confidence interval for the average lifespan of all light bulbs produced by the company.
Solution:
This problem requires applying the CLT to construct a confidence interval. Since the sample size is large (n=100), we can assume the sampling distribution of the mean is approximately normal.
- Point Estimate: 1000 hours
- Standard Error: 100/√100 = 10 hours
- Critical Value (for 95% confidence): Approximately 1.96 (from the z-table)
- Margin of Error: 1.96 * 10 = 19.6 hours
- Confidence Interval: 1000 ± 19.6 or (980.4, 1019.6) hours
Therefore, we are 95% confident that the true average lifespan of the company's light bulbs lies between 980.4 and 1019.6 hours.
Example 3: Impact of Sample Size
Two researchers are studying the average income of a city's residents. Researcher A takes a sample of 100 residents, while Researcher B takes a sample of 1000 residents. Both researchers calculate 95% confidence intervals for the average income. Which researcher's confidence interval will be narrower?
Solution:
Researcher B's confidence interval will be narrower. A larger sample size (1000) leads to a smaller standard error and thus a smaller margin of error, resulting in a narrower confidence interval. Larger sample sizes provide more precise estimates.
Mastering the MCQ Part C: Advanced Strategies
- Practice, Practice, Practice: The key to success is consistent practice. Work through numerous problems, focusing on understanding the underlying concepts rather than just memorizing formulas.
- Visualize: Drawing diagrams and sketching sampling distributions can greatly aid in understanding the problem.
- Break Down Complex Problems: Deconstruct complex questions into smaller, manageable parts. Identify the key concepts being tested and address them individually.
- Understand Assumptions: Pay close attention to the assumptions made in applying the CLT. For example, the assumption of independence is crucial.
- Review Formulas: While conceptual understanding is paramount, ensure you're comfortable with the relevant formulas for calculating standard errors, margins of error, and confidence intervals.
- Utilize Practice Exams: Official AP Statistics practice exams and released questions provide invaluable experience with the format and style of the exam questions.
By mastering the fundamentals of sampling distributions, the Central Limit Theorem, and confidence intervals, and by employing these strategic approaches, you can significantly improve your performance on AP Statistics Unit 7 Progress Check MCQ Part C and achieve success on the AP exam. Remember, consistent effort and a deep understanding of the underlying concepts are essential for tackling these challenging questions.
Latest Posts
Latest Posts
-
What Is A Good Reason To Practice Recognizing Stereotypes
Apr 22, 2025
-
The Philosophy Of Zero Defects Is
Apr 22, 2025
-
How Would One Gain Tacit Knowledge
Apr 22, 2025
-
Study Guide Dna Rna And Protein Synthesis Answer Key
Apr 22, 2025
-
Which Best Describes The Perceptual Communication Model Of Visual Communications
Apr 22, 2025
Related Post
Thank you for visiting our website which covers about Ap Statistics Unit 7 Progress Check Mcq Part C . We hope the information provided has been useful to you. Feel free to contact us if you have any questions or need further assistance. See you next time and don't miss to bookmark.