You Are Interested In Estimating The Mean Of A Population
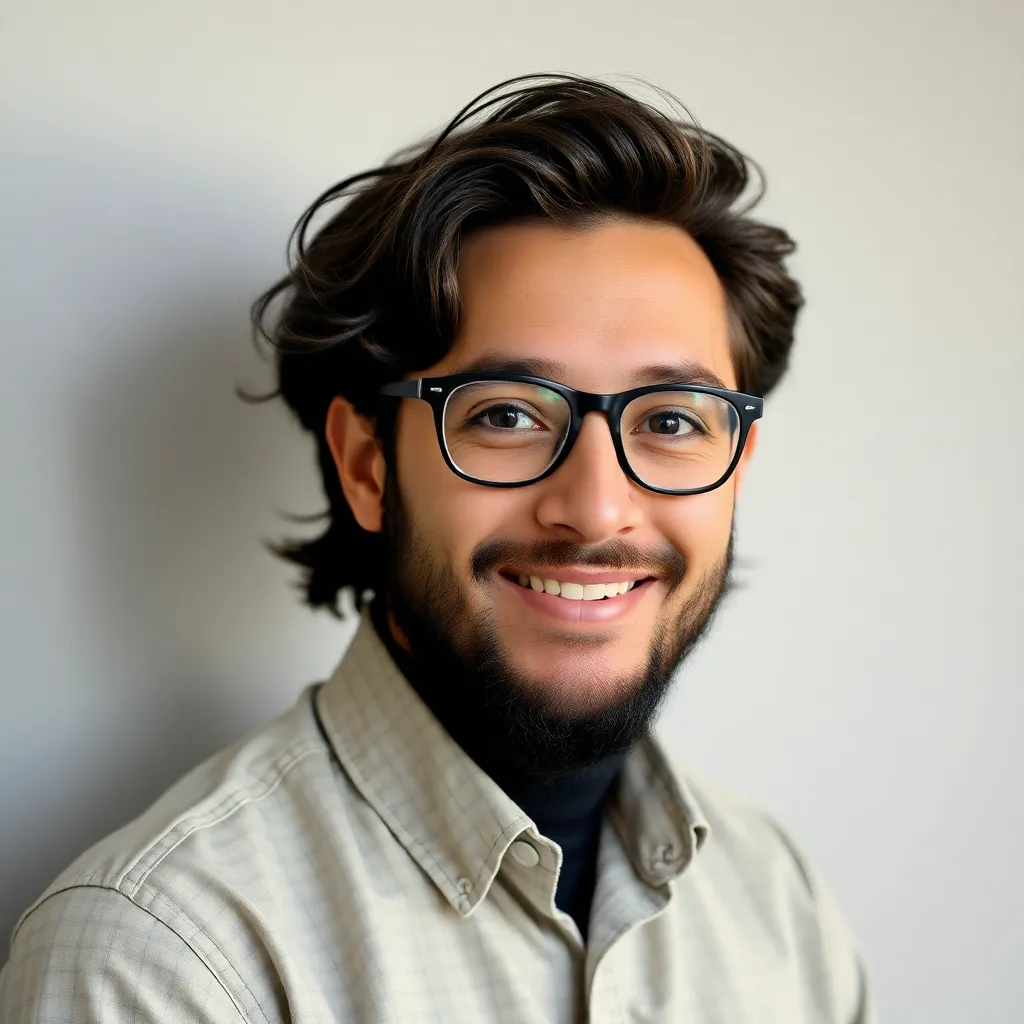
New Snow
Apr 17, 2025 · 6 min read

Table of Contents
Estimating the Mean of a Population: A Comprehensive Guide
Estimating the population mean is a fundamental concept in statistics, crucial for drawing inferences about a larger group based on a smaller sample. This process is used extensively across various fields, from market research analyzing customer preferences to medical studies evaluating treatment efficacy. Understanding the methods involved, their assumptions, and their limitations is vital for accurate and reliable results. This comprehensive guide will delve into the intricacies of estimating the population mean, exploring different techniques and their applications.
Understanding the Concept of Population Mean
The population mean, denoted by μ (mu), represents the average value of a variable across an entire population. A population can be anything from the height of all adult women in a country to the lifespan of a specific type of lightbulb. Determining the true population mean often proves impractical or impossible due to the sheer size and accessibility of many populations. This is where sampling and estimation come into play.
We obtain a sample – a subset of the population – and use its characteristics to estimate the population mean. The sample mean, denoted by x̄ (x-bar), is calculated by summing all the values in the sample and dividing by the number of observations (n). While x̄ provides a point estimate of μ, it's unlikely to be exactly equal to the true population mean due to inherent sampling variability.
Methods for Estimating the Population Mean
Several methods exist for estimating the population mean, each with its strengths and weaknesses. The choice of method often depends on factors such as the sample size, the nature of the data, and the level of confidence required.
1. Point Estimation
The simplest method involves calculating the sample mean (x̄). This serves as a point estimate of the population mean. However, a point estimate alone doesn't convey the uncertainty associated with the estimate. It doesn't tell us how likely it is that our sample mean is close to the true population mean.
Example: Imagine surveying 100 customers about their satisfaction with a product, with an average rating of 4.2 out of 5. 4.2 is the point estimate of the average customer satisfaction for the entire population. This doesn't, however, give us any idea of how certain we are that the true population mean lies close to 4.2.
2. Interval Estimation (Confidence Intervals)
To address the limitations of point estimation, we use interval estimation. This involves constructing a confidence interval, which provides a range of values within which the true population mean is likely to fall. The confidence interval is expressed as:
x̄ ± Margin of Error
The margin of error quantifies the uncertainty associated with the point estimate. A larger margin of error indicates greater uncertainty. The margin of error depends on several factors including:
- Sample size (n): Larger samples lead to smaller margins of error. Larger samples provide more information, leading to more precise estimations.
- Standard deviation (σ or s): A larger standard deviation indicates more variability in the data, resulting in a larger margin of error. The standard deviation represents the spread or dispersion of the data around the mean.
- Confidence level: This represents the probability that the true population mean lies within the calculated interval. Common confidence levels are 90%, 95%, and 99%. A higher confidence level leads to a wider interval.
The formula for the margin of error often uses the standard error of the mean (SEM), which is calculated as:
SEM = σ / √n
(if population standard deviation σ is known)
SEM = s / √n
(if population standard deviation is unknown and sample standard deviation s is used)
Where:
- σ is the population standard deviation
- s is the sample standard deviation
- n is the sample size
The choice between using σ or s depends on whether the population standard deviation is known. In most real-world scenarios, the population standard deviation is unknown, and we use the sample standard deviation as an estimate.
When using the sample standard deviation (s), the appropriate distribution to use for calculating the confidence interval is the t-distribution. The t-distribution accounts for the added uncertainty associated with estimating the population standard deviation from the sample data. The t-distribution approaches the normal distribution as the sample size increases.
Example: Let's assume a sample of 100 customers yielded a mean satisfaction rating of 4.2 (x̄) with a sample standard deviation of 0.8 (s). For a 95% confidence level, and using a t-value of approximately 1.98 (obtained from a t-table with 99 degrees of freedom), the margin of error would be:
Margin of Error = 1.98 * (0.8 / √100) ≈ 0.158
Therefore, the 95% confidence interval would be:
4.2 ± 0.158 = (4.042, 4.358)
This means we are 95% confident that the true population mean customer satisfaction rating lies between 4.042 and 4.358.
Assumptions and Limitations
The accuracy and validity of the population mean estimation heavily rely on several key assumptions:
- Random Sampling: The sample must be randomly selected from the population. Non-random sampling can introduce bias, leading to inaccurate estimations.
- Independence of Observations: Each observation in the sample must be independent of the others. This means that the value of one observation should not influence the value of another.
- Normality (for smaller sample sizes): For smaller sample sizes (generally less than 30), the data should approximately follow a normal distribution. If the data is significantly non-normal, other estimation methods may be required. For larger sample sizes, the central limit theorem helps ensure that the sampling distribution of the mean is approximately normal, even if the underlying population is not normally distributed.
- No outliers: Outliers, extreme values that are far from the rest of the data, can disproportionately affect the sample mean and standard deviation, leading to unreliable estimates.
Advanced Techniques
For more complex situations, more advanced techniques might be necessary:
- Stratified Sampling: Dividing the population into subgroups (strata) and then randomly sampling from each stratum. This is useful when the population is heterogeneous and ensures representation from all subgroups.
- Cluster Sampling: Dividing the population into clusters and then randomly selecting a few clusters to sample. This is cost-effective when the population is geographically dispersed.
- Weighted Average: Assigning different weights to observations based on their importance or relevance. This is useful when some observations are more informative than others.
- Bayesian Estimation: Incorporating prior knowledge or beliefs about the population mean into the estimation process. This approach uses Bayes' theorem to update the estimate as new data becomes available.
- Bootstrapping: A resampling technique that involves creating many samples from the original sample by repeatedly drawing with replacement. This allows for the estimation of the sampling distribution and the construction of confidence intervals even when assumptions of normality are not met.
Conclusion
Estimating the population mean is a critical task in statistical inference, enabling us to draw meaningful conclusions about large populations based on smaller samples. Understanding the different methods, their assumptions, and their limitations is essential for the accurate and responsible use of statistical data. The choice of method depends heavily on the specific context, the nature of the data, and the desired level of precision and confidence. By carefully considering these factors and selecting appropriate techniques, researchers can obtain reliable estimates and draw valid inferences about the population mean. Remember to always critically evaluate your data and assumptions to ensure the robustness of your estimations. The pursuit of accurate population mean estimation is a cornerstone of evidence-based decision making across numerous fields.
Latest Posts
Latest Posts
-
Which Of The Following Is An Example Of Collective Behavior
Apr 19, 2025
-
Dr Kettlewell Predicted That Clean Forests Would Have
Apr 19, 2025
-
The Opportunity Cost Of An Activity Is
Apr 19, 2025
-
Diabetes Type 1 Hesi Case Study
Apr 19, 2025
-
Where Should Staff Members Eat Drink Smoke Or Chew Gum
Apr 19, 2025
Related Post
Thank you for visiting our website which covers about You Are Interested In Estimating The Mean Of A Population . We hope the information provided has been useful to you. Feel free to contact us if you have any questions or need further assistance. See you next time and don't miss to bookmark.