Which Of The Following Statements About Models Is Correct
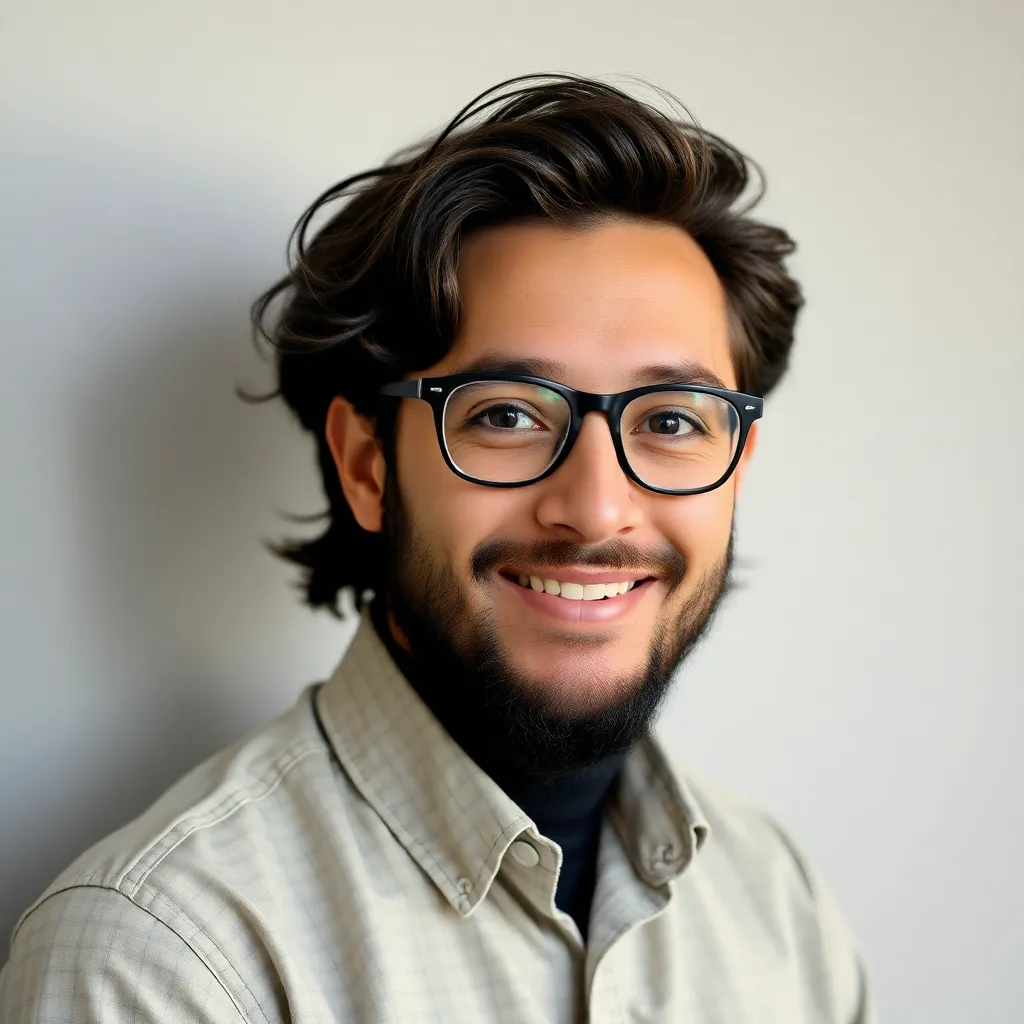
New Snow
Apr 26, 2025 · 5 min read

Table of Contents
Which of the Following Statements About Models is Correct? A Deep Dive into Model Types and Applications
Understanding models is crucial across numerous disciplines. Whether you're discussing scientific theories, business strategies, or even everyday decision-making, models provide simplified representations of complex realities. But with such diverse applications, it's essential to clarify what constitutes a "correct" model. This article delves into the nuances of model accuracy, utility, and applicability, exploring various model types and addressing the complexities inherent in evaluating their correctness.
Defining "Correctness" in Models
The term "correct" when applied to models is inherently subjective and context-dependent. A model isn't necessarily judged as simply "correct" or "incorrect" in a binary sense. Instead, we evaluate its correctness based on its purpose, its accuracy, and its limitations.
1. Purpose-Driven Correctness:
A model's "correctness" is first and foremost determined by how well it serves its intended purpose. A model designed to predict short-term stock fluctuations might be considered "correct" if it accurately forecasts trends within a specific timeframe, even if it fails to predict long-term market behavior. Conversely, a model aiming to forecast long-term climate change would need to demonstrate accuracy over extended periods, even if it struggles with short-term variations. Therefore, the intended use case dictates the criteria for evaluating a model's success.
2. Accuracy and Fidelity:
The accuracy of a model refers to how closely it reflects the real-world phenomenon it represents. High accuracy signifies a strong correlation between the model's predictions and observed data. However, achieving perfect accuracy is often unattainable, particularly when dealing with complex systems. Instead, we strive for sufficient accuracy for the intended application.
Model fidelity, a related concept, assesses the level of detail and complexity captured in the model. A highly faithful model includes numerous variables and nuanced interactions, offering a richer representation of reality. However, highly faithful models can be computationally expensive and challenging to interpret. The choice between fidelity and simplicity involves a trade-off, optimizing for the level of detail required for the specific task.
3. Limitations and Assumptions:
Every model relies on simplifying assumptions, inevitably abstracting away certain aspects of reality. Acknowledging these limitations is crucial to judging a model's "correctness." A model's correctness is not diminished by its limitations, provided these limitations are clearly identified and understood. Transparency about assumptions and constraints is essential for responsible model usage.
Types of Models and Their Evaluation
Numerous model types exist, each with its unique strengths and limitations. Understanding these differences is key to selecting the appropriate model for a given task and evaluating its "correctness" appropriately.
1. Mathematical Models:
Mathematical models utilize mathematical equations and relationships to represent phenomena. These models are often used in physics, engineering, and finance. Evaluating their correctness involves comparing model predictions to empirical data and assessing the statistical significance of any discrepancies. Parameter estimation and model validation techniques are crucial in evaluating the accuracy of mathematical models.
Examples: Newton's Law of Universal Gravitation, the Black-Scholes model for option pricing, epidemiological models for disease spread.
2. Statistical Models:
Statistical models employ statistical techniques to analyze data and make inferences about underlying populations. These models are widely used in various fields, from social sciences to healthcare. Assessing the correctness of statistical models involves considering the model's goodness-of-fit, the significance of coefficients, and the validity of underlying assumptions. Hypothesis testing and confidence intervals play a key role in evaluating the reliability of statistical inferences.
Examples: Linear regression models, logistic regression models, time series models, Bayesian models.
3. Computational Models:
Computational models use computer simulations to explore complex systems that are difficult or impossible to analyze using analytical methods. These models are widely used in climate science, fluid dynamics, and material science. Evaluating their correctness involves comparing simulation outputs to experimental data, assessing computational accuracy, and verifying the model's stability and convergence. Grid resolution, time steps, and numerical methods significantly influence the accuracy of computational models.
Examples: Climate models, fluid dynamics simulations, finite element analysis models.
4. Conceptual Models:
Conceptual models are abstract representations that focus on the relationships between key components of a system rather than precise quantitative predictions. These models are often used in systems thinking, design thinking, and strategic planning. Evaluating the correctness of conceptual models involves assessing their ability to clarify complex relationships, identify key drivers, and guide decision-making. The criteria for judging correctness are largely qualitative, focused on utility and clarity.
Examples: Systems diagrams, flow charts, mental models, causal loop diagrams.
Factors Influencing Model Correctness
Several factors can influence a model's perceived "correctness":
-
Data Quality: Garbage in, garbage out. The accuracy of any model is critically dependent on the quality, relevance, and completeness of the input data. Inaccurate or biased data inevitably lead to inaccurate model predictions.
-
Model Complexity: More complex models can capture greater detail but are more prone to overfitting, meaning the model fits the training data too well but performs poorly on new, unseen data. Simplicity, therefore, often trumps complexity, especially when data is limited.
-
Assumptions and Simplifications: Every model relies on simplifying assumptions that abstract away aspects of reality. Understanding these assumptions is crucial to interpreting model results and recognizing potential limitations.
-
Validation and Testing: Rigorous testing and validation procedures are crucial to assess a model's performance and identify potential biases or limitations. Techniques like cross-validation, holdout samples, and blind testing are essential in evaluating model robustness.
-
Interpretability and Explainability: The ease with which model results can be interpreted and understood significantly impacts their usefulness and acceptance. Opaque "black box" models, while potentially highly accurate, can be problematic if their predictions cannot be understood or explained.
Conclusion: Beyond Binary Judgments of Correctness
Assessing the "correctness" of a model is not a straightforward binary classification. Instead, it requires a nuanced understanding of the model's purpose, limitations, accuracy, and the context of its application. By considering the factors discussed above, we can move beyond simple "correct" or "incorrect" evaluations and instead engage in a more critical and informed assessment of model utility and reliability. The key is to evaluate a model in relation to its intended use, acknowledge its limitations, and utilize appropriate validation techniques to ensure its reliability and trustworthiness. A model should be judged not solely on its accuracy, but on its usefulness in providing insights and informing decision-making within the specific context of its application.
Latest Posts
Latest Posts
-
Interest Rates Of Non Bank Credit Alternatives
Apr 26, 2025
-
The Mutation Results In A Of Function
Apr 26, 2025
-
The Core Mechanisms Of Group Process And Change Include
Apr 26, 2025
-
Which Of The Following Is Something Antibodies Do Not Do
Apr 26, 2025
-
Ap Physics 1 Unit 1 Progress Check Mcq Pdf
Apr 26, 2025
Related Post
Thank you for visiting our website which covers about Which Of The Following Statements About Models Is Correct . We hope the information provided has been useful to you. Feel free to contact us if you have any questions or need further assistance. See you next time and don't miss to bookmark.