Which Of The Following Is Not A Level Of Measurement
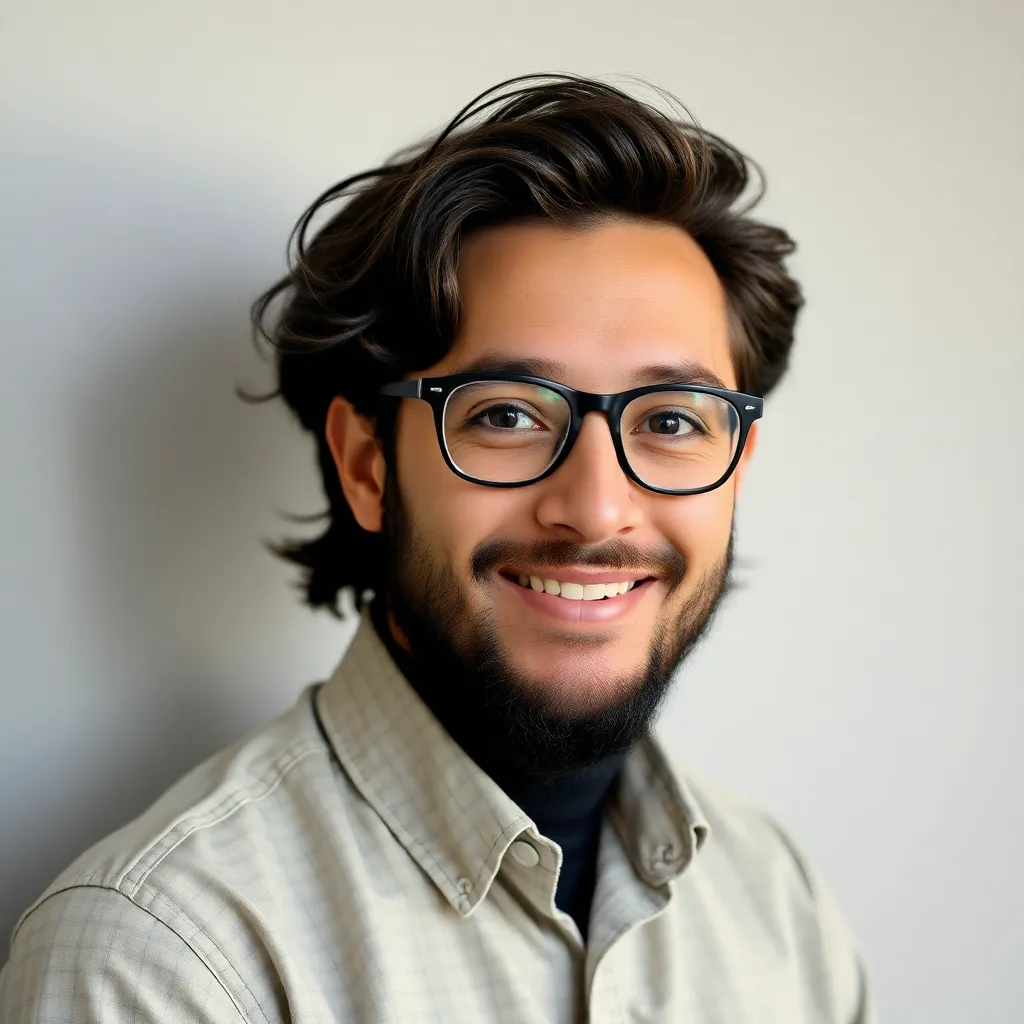
New Snow
Apr 22, 2025 · 7 min read

Table of Contents
Which of the Following is NOT a Level of Measurement? Understanding Measurement Scales in Research
Measurement is the cornerstone of any robust research study. Understanding the different levels of measurement is crucial for selecting appropriate statistical analyses and accurately interpreting results. This article will delve into the four fundamental levels of measurement – nominal, ordinal, interval, and ratio – explaining their characteristics and highlighting the key distinctions between them. We'll also explore why certain types of data might not fit neatly into these categories, emphasizing the importance of choosing the right scale for your research objectives.
The Four Fundamental Levels of Measurement
Before we can determine what isn't a level of measurement, it's vital to understand the four established levels:
1. Nominal Level of Measurement
This is the most basic level. Nominal data categorizes variables into distinct groups without any inherent order or ranking. Think of it as simply assigning names or labels to different categories.
Examples:
- Gender: Male, Female, Other
- Eye Color: Brown, Blue, Green, Hazel
- Marital Status: Single, Married, Divorced, Widowed
Key Characteristics:
- Qualitative: Deals with descriptive characteristics rather than numerical values.
- Mutually Exclusive: Each observation belongs to only one category.
- Exhaustive: All possible categories are included.
- No inherent order: There's no meaningful way to rank the categories. "Brown" isn't inherently "better" or "worse" than "Blue."
You can't perform mathematical operations (like calculating an average) on nominal data. The only calculations that are meaningful are frequencies (how many observations fall into each category) and percentages.
2. Ordinal Level of Measurement
Ordinal data takes us a step further. It categorizes variables into ranked groups, establishing a meaningful order or hierarchy. However, the distances between the categories are not necessarily equal.
Examples:
- Education Level: High School, Bachelor's Degree, Master's Degree, PhD
- Customer Satisfaction: Very Satisfied, Satisfied, Neutral, Dissatisfied, Very Dissatisfied
- Ranking in a Competition: 1st place, 2nd place, 3rd place
Key Characteristics:
- Ranked Categories: Categories have a clear order, but the differences between them aren't necessarily consistent. The difference between "High School" and "Bachelor's Degree" is not the same as the difference between "Master's Degree" and "PhD."
- Qualitative and Quantitative Aspects: While possessing order, the numbers assigned are often arbitrary.
3. Interval Level of Measurement
Interval data goes beyond ordinal data by introducing equal intervals between categories. This allows for meaningful comparisons of differences between data points. However, interval scales lack a true zero point.
Examples:
- Temperature in Celsius or Fahrenheit: The difference between 20°C and 30°C is the same as the difference between 30°C and 40°C. However, 0°C doesn't represent the absence of temperature.
- Years: The difference between 2000 and 2010 is the same as the difference between 2010 and 2020. Year 0 doesn't indicate the absence of time.
- Scores on some standardized tests: While the scores represent a ranking, the intervals between scores can be considered equal.
Key Characteristics:
- Equal Intervals: The distance between consecutive points is constant.
- Arbitrary Zero Point: Zero doesn't represent the absence of the measured attribute.
4. Ratio Level of Measurement
Ratio data is the most informative level. It possesses all the characteristics of interval data, plus a true zero point. This true zero signifies the absence of the measured attribute.
Examples:
- Height: A height of 0cm means no height.
- Weight: A weight of 0kg means no weight.
- Income: An income of $0 means no income.
- Age: An age of 0 indicates a newborn.
Key Characteristics:
- Equal Intervals: The distance between consecutive points is constant.
- True Zero Point: Zero represents the absence of the attribute being measured.
- Ratios are meaningful: You can meaningfully say that someone who is 180cm tall is twice as tall as someone who is 90cm tall.
What is NOT a Level of Measurement?
While the four levels outlined above are widely accepted, it's crucial to recognize that not all data perfectly aligns with these categories. Data that lacks clear categorization or fails to meet the criteria of any of these scales falls outside these established levels.
Here are some scenarios that illustrate this:
-
Data with inconsistent intervals: If a scale purports to be interval but the intervals between categories are uneven or inconsistent, it doesn't truly fit the interval level. For example, a satisfaction scale with wildly disparate points (1-very dissatisfied, 2-somewhat dissatisfied, 3-neutral, 4-very satisfied, 5-extremely, incredibly satisfied) lacks the equal spacing needed for interval level data. Such a scale would be better treated as ordinal.
-
Unclear or ambiguous categories: If the categories used for measurement are vague or overlapping, it undermines the clarity and precision needed for any of the four levels. For instance, a survey question on "political affiliation" that includes categories like "liberal," "conservative," and "moderate" may suffer from ambiguity as these terms can be defined differently by individuals.
-
Data with subjective interpretation: When data relies heavily on subjective judgments or opinions without a standardized scoring mechanism, assigning it to a specific level becomes difficult. For example, responses to open-ended survey questions are often rich in qualitative data but don't automatically fit into a numerical scale. These usually need further coding and categorization before they can be analyzed quantitatively.
-
Data that lacks a clear conceptualization of the variable: Sometimes, the researcher’s definition of the variable itself is fuzzy, making appropriate measurement challenging. Without a clear understanding of what is being measured, fitting the data into one of the four levels is an exercise in futility.
Dealing with Data That Doesn't Fit:
When encountering data that doesn't neatly fit into the standard levels of measurement, several strategies can be employed:
-
Refine the Measurement Instrument: If the issue lies in the measurement tool itself, revise it to create clearer, more precise categories and consistent intervals.
-
Re-categorize the Data: This could involve grouping similar data points to create clearer categories or re-coding responses to align with a specific level of measurement.
-
Use Qualitative Analysis Techniques: If the data inherently lacks numerical properties, embrace qualitative analysis methods such as thematic analysis or grounded theory to uncover patterns and insights.
-
Consider Mixed-Methods Approach: Combine quantitative and qualitative methods to gain a more comprehensive understanding of the data. The quantitative component might involve assigning data to the most appropriate level based on its properties, while qualitative analysis helps to illuminate the nuances and complexities.
-
Transforming Data: In some cases, it is possible to transform the data into a more appropriate level. For example, ordinal data might be transformed into numerical rankings to facilitate certain types of analysis.
The Importance of Selecting the Right Level of Measurement
Choosing the correct level of measurement is paramount for several reasons:
-
Appropriate Statistical Analysis: Different statistical techniques require different levels of measurement. Using inappropriate techniques can lead to inaccurate or misleading results.
-
Valid Interpretation of Results: Accurate interpretation relies on understanding the limitations of the chosen level of measurement. This informs how the results are presented and discussed.
-
Reproducibility of Findings: Clear specification of the measurement level ensures that other researchers can replicate the study and verify the results.
-
Enhanced Research Rigor: Using the correct measurement levels enhances the overall scientific rigor and credibility of the research.
Conclusion
Understanding the different levels of measurement – nominal, ordinal, interval, and ratio – is essential for conducting rigorous and meaningful research. While these four levels provide a valuable framework, it's crucial to recognize that not all data will perfectly conform to them. Knowing how to handle data that doesn't easily fit into these categories, using appropriate analytical techniques, and being transparent about the limitations of the chosen measurement level contribute significantly to the quality and trustworthiness of the research findings. Always strive for clarity and precision in defining variables and choosing measurement scales that appropriately capture the complexity of your research questions.
Latest Posts
Latest Posts
-
Indicate Whether The Following Statements Are True Or False
Apr 22, 2025
-
Who Facilitates The Operational Period Briefing
Apr 22, 2025
-
What Is The First Step In A Disaster Recovery Effort
Apr 22, 2025
-
Todas As Coisas Que Eu Te Escreveria Se Pudesse Pdf
Apr 22, 2025
-
What Is The Correct Order Of The Loss Limitation Rules
Apr 22, 2025
Related Post
Thank you for visiting our website which covers about Which Of The Following Is Not A Level Of Measurement . We hope the information provided has been useful to you. Feel free to contact us if you have any questions or need further assistance. See you next time and don't miss to bookmark.