Which Normal Distribution Has The Greatest Standard Deviation
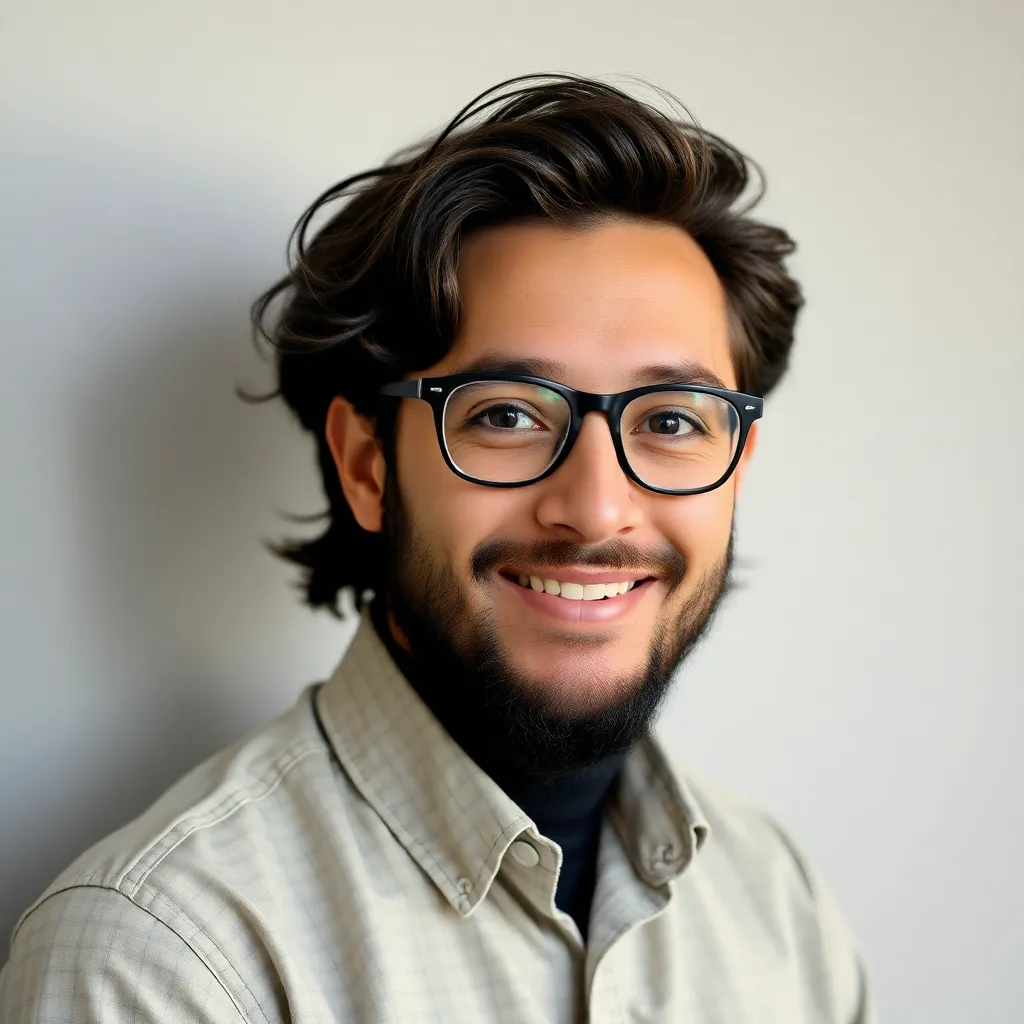
New Snow
Apr 19, 2025 · 5 min read

Table of Contents
Which Normal Distribution Has the Greatest Standard Deviation? Understanding the Nature of the Standard Deviation in Normal Distributions
The question, "Which normal distribution has the greatest standard deviation?" is a bit of a trick question. The answer isn't a specific distribution, but rather a concept: there is no limit to how large the standard deviation of a normal distribution can be. This understanding hinges on grasping the fundamental properties of the normal distribution and the standard deviation itself.
Understanding the Normal Distribution
The normal distribution, also known as the Gaussian distribution, is a ubiquitous probability distribution in statistics. Its bell-shaped curve is characterized by its symmetry around the mean (average) and its spread, which is quantified by the standard deviation. Many natural phenomena, such as height, weight, and IQ scores, approximately follow a normal distribution.
A normal distribution is completely defined by two parameters:
- Mean (μ): This represents the center of the distribution, the point of highest probability density.
- Standard Deviation (σ): This measures the spread or dispersion of the data around the mean. A larger standard deviation indicates a wider spread, while a smaller standard deviation indicates a narrower spread.
The normal distribution is mathematically described by the probability density function:
f(x) = (1/σ√(2π)) * exp(-(x-μ)²/(2σ²))
Where:
f(x)
is the probability density at a given value x.μ
is the mean.σ
is the standard deviation.exp()
denotes the exponential function.π
is the mathematical constant pi (approximately 3.14159).
What is Standard Deviation?
The standard deviation is a critical measure of variability. It quantifies how much the individual data points deviate from the mean. A higher standard deviation indicates greater variability, meaning the data points are more spread out. Conversely, a lower standard deviation indicates less variability, meaning the data points are clustered closer to the mean.
Calculating the Standard Deviation:
The standard deviation (σ) is calculated using the following formula for a population:
σ = √[ Σ(xi - μ)² / N ]
Where:
xi
represents each individual data point.μ
is the population mean.N
is the total number of data points in the population.
For a sample, a slightly modified formula is used, incorporating Bessel's correction:
s = √[ Σ(xi - x̄)² / (n - 1) ]
Where:
xi
represents each individual data point in the sample.x̄
is the sample mean.n
is the total number of data points in the sample.
Why There's No Largest Standard Deviation for a Normal Distribution
The key to understanding why there's no largest standard deviation for a normal distribution lies in the nature of the distribution itself. The normal distribution is defined for all real numbers from negative infinity to positive infinity (-∞, +∞). This means the data points can theoretically spread infinitely far from the mean. Consequently, the standard deviation, which measures this spread, can also be arbitrarily large.
There is no inherent upper bound on how much the data can deviate from the mean within the context of a normal distribution. You can always conceive of a normal distribution with a larger standard deviation than any previously considered distribution.
Practical Implications and Examples
While theoretically the standard deviation can be infinite, in practice, we rarely encounter distributions with extremely large standard deviations in real-world scenarios. The data usually exhibit some degree of natural constraint. For example:
- Height: While height approximately follows a normal distribution, there are physical limitations to how tall a human can realistically grow. The standard deviation will reflect this constraint.
- Temperature: While temperature data might follow a normal distribution within a specific region and time period, the physical limits of temperature (absolute zero and beyond) will practically limit the spread and, consequently, the standard deviation.
- Test Scores: Exam scores often show a roughly normal distribution, but the scoring system inherently limits the range of scores, restricting the possible standard deviation.
Comparing Normal Distributions with Different Standard Deviations
Consider two normal distributions:
- Distribution A: Mean (μ) = 50, Standard Deviation (σ) = 5
- Distribution B: Mean (μ) = 50, Standard Deviation (σ) = 15
Distribution B has a much larger standard deviation than Distribution A. This means the data points in Distribution B are significantly more spread out around the mean of 50 compared to those in Distribution A. The bell curve of Distribution B will be wider and flatter than that of Distribution A. The probability of observing extreme values will be higher in Distribution B.
Visualizing the Concept
Imagine graphing several normal distributions with increasing standard deviations but the same mean. You'll see the bell curve getting progressively wider and flatter. As the standard deviation increases, the peak of the curve becomes lower, and the tails of the distribution extend further out. There's no point where this widening stops – it continues indefinitely.
The Importance of Standard Deviation in Statistical Analysis
The standard deviation is a cornerstone of statistical analysis. It plays a crucial role in:
- Hypothesis testing: Determining the significance of observed differences between groups.
- Confidence intervals: Estimating the range within which a population parameter likely lies.
- Data interpretation: Understanding the variability and spread of data.
- Quality control: Monitoring and evaluating process variability.
- Risk assessment: Quantifying uncertainty and variability in financial models or other applications.
Conclusion
In summary, there is no normal distribution with the "greatest" standard deviation. The standard deviation of a normal distribution can be arbitrarily large. This understanding is fundamental to appreciating the flexibility and wide applicability of the normal distribution in statistical modeling and analysis. While real-world data often exhibits practical limitations that restrict the observed standard deviation, the theoretical possibility of an infinitely large standard deviation remains a key characteristic of the normal distribution. Understanding this theoretical limit is crucial for proper interpretation and application of statistical concepts.
Latest Posts
Latest Posts
-
Cell Membrane Bubble Lab Student Analysis
Apr 19, 2025
-
Luthan Company Uses A Plantwide Predetermined
Apr 19, 2025
-
11 5 11 Secure A Home Wireless Network
Apr 19, 2025
-
The Field Of Nutrition Is Defined By Which Three Elements
Apr 19, 2025
-
Which Best Describes The Sensory Communication Model Of Visual Communications
Apr 19, 2025
Related Post
Thank you for visiting our website which covers about Which Normal Distribution Has The Greatest Standard Deviation . We hope the information provided has been useful to you. Feel free to contact us if you have any questions or need further assistance. See you next time and don't miss to bookmark.