Which Measure Of Variation Is Most Sensitive To Extreme Values
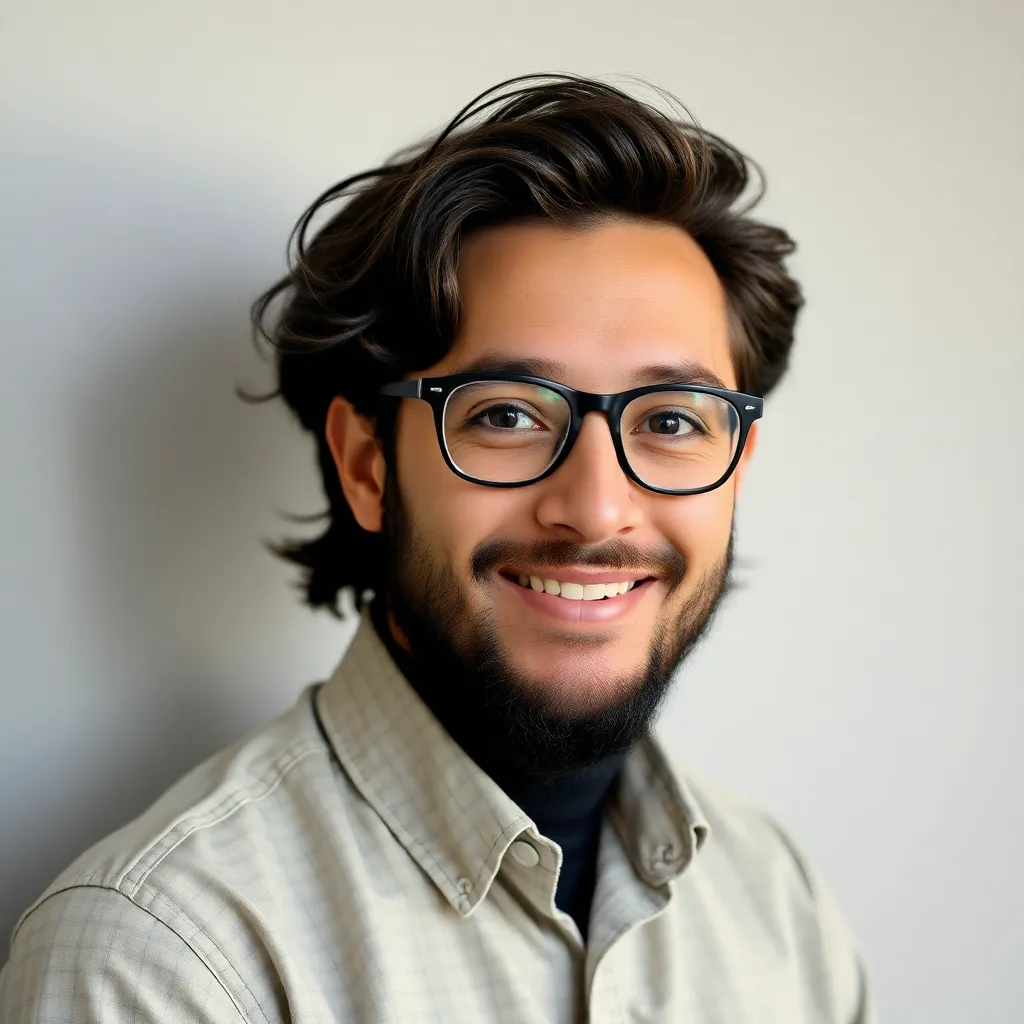
New Snow
Apr 24, 2025 · 6 min read

Table of Contents
Which Measure of Variation is Most Sensitive to Extreme Values?
Understanding data variability is crucial in statistics. Variability, or dispersion, describes how spread out a dataset is. Several measures quantify this spread, each with its strengths and weaknesses. This article delves deep into the sensitivity of different measures of variation to extreme values, ultimately determining which is most susceptible to outliers and why this matters in your analysis.
Understanding Measures of Variation
Before diving into sensitivity, let's review common measures of variation:
1. Range
The range is the simplest measure. It's calculated by subtracting the smallest value from the largest value in a dataset. While easy to compute, the range is highly sensitive to outliers. A single extreme value can drastically inflate the range, misrepresenting the typical spread of the data.
Example: Consider the dataset: 2, 4, 6, 8, 10. The range is 10 - 2 = 8. Now, add an outlier: 100. The range becomes 100 - 2 = 98. The outlier dramatically altered the range.
2. Interquartile Range (IQR)
The interquartile range (IQR) is more robust to outliers than the range. It represents the spread of the middle 50% of the data. The IQR is calculated as the difference between the third quartile (Q3) and the first quartile (Q1). Quartiles divide the sorted data into four equal parts.
Example: For the dataset 2, 4, 6, 8, 10, Q1 = 4 and Q3 = 8. The IQR = 8 - 4 = 4. Adding the outlier 100 changes the quartiles, but the impact is less dramatic than on the range. The IQR provides a more stable measure of spread, less swayed by extreme values.
3. Variance
The variance measures the average squared deviation of each data point from the mean. It’s calculated by summing the squared differences between each data point and the mean, then dividing by the number of data points (or N-1 for sample variance). Because it squares the deviations, larger deviations (caused by outliers) have a disproportionately large impact on the variance.
Example: The variance of the dataset 2, 4, 6, 8, 10 is relatively small. However, adding 100 significantly increases the variance because the squared difference (100 - mean)^2 is substantial.
4. Standard Deviation
The standard deviation is the square root of the variance. Like the variance, it is sensitive to extreme values. Outliers significantly influence the standard deviation, making it a less robust measure when dealing with datasets containing extreme values. The standard deviation is expressed in the same units as the original data, making it easier to interpret than variance.
Example: Similar to variance, the standard deviation of {2, 4, 6, 8, 10} is relatively small. However, including 100 substantially inflates the standard deviation, reflecting the influence of the outlier.
5. Mean Absolute Deviation (MAD)
The mean absolute deviation (MAD) measures the average absolute deviation of each data point from the mean. It’s calculated by summing the absolute differences between each data point and the mean, then dividing by the number of data points. While less sensitive than the variance and standard deviation, it's still affected by outliers, albeit less dramatically.
Example: The MAD is less affected by the outlier than the variance or standard deviation but is still influenced. The absolute differences are summed, and while the outlier contributes a large absolute difference, it doesn't have the same disproportionate impact as squaring the difference in variance and standard deviation.
Which Measure is Most Sensitive?
Of the measures discussed, the range is unequivocally the most sensitive to extreme values. A single outlier can completely distort the range, making it an unreliable measure of variation when outliers are present. The variance and standard deviation are also highly sensitive due to the squaring of deviations. While the MAD and IQR are more robust, they're still affected to some degree.
Why Sensitivity Matters
The sensitivity of these measures to extreme values has significant implications:
-
Misleading Descriptive Statistics: Using sensitive measures with outlier-prone datasets can create a misleading picture of the data's typical spread. This can lead to inaccurate conclusions.
-
Impact on Inferential Statistics: Many inferential statistical tests (like t-tests and ANOVA) rely on assumptions about data distribution, including assumptions about variance. Outliers can violate these assumptions, impacting the validity of the results.
-
Data Cleaning and Preprocessing: Understanding sensitivity helps determine the appropriateness of data cleaning techniques. Should outliers be removed, transformed (e.g., winsorizing), or left as is? The choice depends on the context, the nature of the outliers, and the chosen measure of variation.
-
Model Building and Robustness: In machine learning and predictive modeling, sensitive measures can negatively impact model performance. Outliers can bias model parameters, leading to poor predictions on unseen data. Robust statistical methods often employ less sensitive measures like the IQR or MAD to develop more resilient models.
Dealing with Outliers and Choosing the Right Measure
The presence of outliers necessitates careful consideration when selecting a measure of variation. There's no one-size-fits-all solution. The best approach depends on:
-
The nature of the outliers: Are they genuine data points, or are they due to measurement error or data entry mistakes?
-
The research question: What aspect of data variability is most relevant to your analysis?
-
The robustness of downstream analyses: Are you using the measure of variation in a context where sensitivity to outliers is particularly problematic (e.g., hypothesis testing)?
Here's a suggested approach:
-
Identify and Investigate Outliers: Use visualization techniques (box plots, scatter plots) and statistical methods (e.g., Z-scores) to identify potential outliers. Investigate the cause of these outliers. Were they caused by errors, or do they represent genuine extreme values within the population you’re studying?
-
Robust Measures for Outlier-Prone Data: If outliers are present and deemed genuine, it is best to consider using robust measures like the IQR or MAD. These measures provide a more stable description of the data's typical spread, less influenced by extreme values.
-
Data Transformation: Consider transforming the data (e.g., using logarithmic or other transformations) to reduce the influence of outliers. This can make the data more amenable to analyses that assume a normal distribution or are sensitive to outliers.
-
Consider Non-parametric Methods: If your data significantly deviates from normality due to outliers, consider non-parametric statistical tests, which don't rely on assumptions about data distribution.
-
Documentation: Clearly document your decisions regarding outlier handling and the chosen measure of variation, justifying your choices based on the characteristics of your dataset and the goals of your analysis.
Conclusion
The range is the most sensitive measure of variation to extreme values. Variance and standard deviation are also highly sensitive due to the squaring of deviations. While the IQR and MAD offer increased robustness, no measure is completely immune to the influence of outliers. Understanding the sensitivity of different measures of variation is crucial for making informed decisions in data analysis and ensuring the reliability and validity of your results. Always carefully consider the context, nature of outliers, and downstream analytical implications when choosing an appropriate measure. Through careful data exploration, robust statistical methods, and transparent documentation, you can navigate the complexities of outlier-prone datasets and obtain more accurate and meaningful insights.
Latest Posts
Latest Posts
-
Robert Schumanns Fantasiestuecke Is What Type Of Work
Apr 24, 2025
-
Write A Slogan Using The Media Technique Of Association
Apr 24, 2025
-
Is What The Firm Does Better Than Anyone Else
Apr 24, 2025
-
In The Mountains Of Brazil Barbacenia Tomentosa
Apr 24, 2025
-
According To The Results Of The Pillbug Experiment
Apr 24, 2025
Related Post
Thank you for visiting our website which covers about Which Measure Of Variation Is Most Sensitive To Extreme Values . We hope the information provided has been useful to you. Feel free to contact us if you have any questions or need further assistance. See you next time and don't miss to bookmark.