What Is The Shape Of The Histogram Below
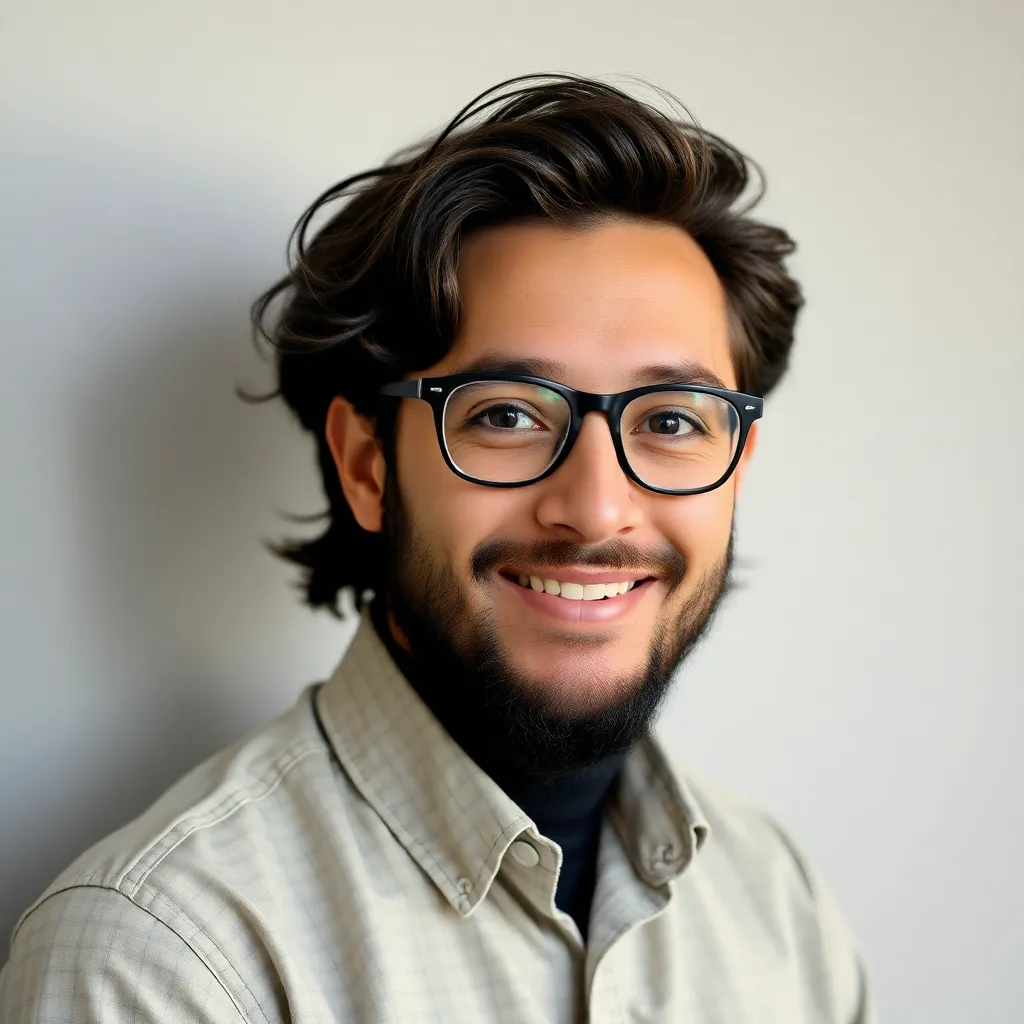
New Snow
Apr 21, 2025 · 6 min read

Table of Contents
Deconstructing the Histogram: Unveiling its Shape and What it Means
Histograms are powerful visual tools used in statistics to represent the distribution of numerical data. Unlike bar charts which represent categorical data, histograms show the frequency distribution of continuous data, grouping values into bins or intervals. The shape of a histogram provides crucial insights into the underlying data, revealing patterns, trends, and potential outliers. Therefore, understanding how to interpret the shape of a histogram is fundamental to data analysis. But what is the shape of the histogram below? Unfortunately, I cannot see the histogram you are referring to. However, I can guide you through the process of analyzing a histogram's shape and interpreting its meaning, enabling you to confidently analyze any histogram you encounter.
This article will cover various histogram shapes, their interpretations, and the implications for data analysis. We'll delve into the characteristics of each shape, including symmetry, skewness, kurtosis, and modality, providing practical examples and explaining how to determine the underlying distribution from the histogram's visual representation. We'll also discuss the importance of context and how additional information can refine your analysis.
Common Histogram Shapes and Their Interpretations
A histogram's shape isn't a rigid classification; it's a descriptive tool to understand the data's distribution. Several common shapes help categorize the data distribution:
1. Symmetrical Histogram:
- Characteristics: A symmetrical histogram is perfectly balanced. A vertical line drawn through the center of the histogram would divide it into two mirror images. The mean, median, and mode are approximately equal and are located at the center of the distribution.
- Interpretation: Symmetrical histograms often suggest a normal distribution or a near-normal distribution. This indicates a common pattern, where data points cluster around a central value, with fewer data points farther away.
- Example: Height measurements of adult women in a specific population would likely show a near-symmetrical histogram.
2. Skewed Histograms:
Skewness describes the asymmetry of a distribution. Histograms can be skewed to the right (positively skewed) or to the left (negatively skewed).
-
Right-Skewed (Positively Skewed):
- Characteristics: The tail on the right side of the histogram is longer than the left. The mean is greater than the median, which is greater than the mode.
- Interpretation: A right-skewed histogram indicates that there are a few very high values (outliers) pulling the mean to the right. The majority of data points are clustered toward the lower end of the range.
- Example: Income distribution in a country often exhibits a right-skewed histogram, with a few high earners pulling the average income up.
-
Left-Skewed (Negatively Skewed):
- Characteristics: The tail on the left side of the histogram is longer than the right. The mean is less than the median, which is less than the mode.
- Interpretation: A left-skewed histogram suggests that there are a few very low values pulling the mean to the left. Most data points are clustered towards the higher end of the range.
- Example: Exam scores on a very easy test might show a left-skewed distribution, with most students scoring high.
3. Unimodal, Bimodal, and Multimodal Histograms:
The modality refers to the number of peaks or modes in a histogram.
- Unimodal: A histogram with a single peak. This indicates that the data clusters around a single central value.
- Bimodal: A histogram with two peaks. This often suggests two distinct subgroups within the data.
- Multimodal: A histogram with more than two peaks, indicating multiple distinct subgroups within the data.
4. Uniform Histogram:
- Characteristics: A uniform histogram shows roughly equal frequencies across all bins. There's no clear central tendency or skewness.
- Interpretation: This suggests a random distribution or that no dominant trend or pattern exists in the data.
- Example: Generating random numbers between 1 and 100 might result in a roughly uniform histogram.
5. Other Shapes and Considerations:
Beyond these fundamental shapes, histograms can exhibit other patterns, including:
- Platykurtic: Flatter than a normal distribution, indicating low variability.
- Leptokurtic: Taller and narrower than a normal distribution, suggesting high variability and potential outliers.
- U-Shaped: Two peaks at the extremes with a low point in the middle, suggesting two distinct subgroups with little overlap.
Analyzing a Histogram: A Step-by-Step Approach
To effectively analyze a histogram, follow these steps:
- Examine the overall shape: Is it symmetrical, skewed to the left or right, or uniform? Note the presence of any distinct peaks (modes).
- Identify the central tendency: Visually estimate the center of the distribution. This can be the approximate mean, median, or mode.
- Assess the spread or variability: Observe the range of values and how the data is dispersed around the central tendency. Is the distribution narrow or wide?
- Look for outliers: Are there any data points that are significantly far from the central tendency?
- Consider the context: The interpretation of the histogram's shape depends heavily on the context of the data. What is being measured? What is the sample size? What are the potential sources of bias or variability?
The Importance of Context and Further Analysis
Interpreting a histogram requires more than just observing its shape. The context of the data is crucial. Consider these points:
- Data Source and Sampling Method: How was the data collected? Was the sample representative of the population? Sampling bias can significantly affect the shape of the histogram.
- Data Transformation: Sometimes, transforming the data (e.g., using a logarithmic transformation) can improve the symmetry and normality of the data, making analysis easier.
- Outliers: Outliers can heavily influence the histogram's shape. Consider whether they are genuine data points or errors. If they are errors, remove them. If genuine, they might represent a significant aspect of your dataset.
- Sample Size: A small sample size may lead to a histogram that doesn’t accurately reflect the true underlying distribution.
Conclusion: Unlocking the Secrets of Your Data
The shape of a histogram is a powerful visual cue that provides valuable insights into the distribution of your data. By understanding the characteristics of different shapes, and by considering the context and nuances of your data, you can effectively interpret a histogram and extract meaningful insights. This ability is an essential skill for anyone working with data, from students to experienced data scientists. Remember to always consider the context, the potential for bias, and the importance of sample size to provide a reliable and accurate interpretation. By combining visual analysis with statistical techniques, you can unlock the secrets of your data and make data-driven decisions with confidence. Remember that this article is a guide. Providing the histogram would enable a much more precise and detailed analysis.
Latest Posts
Latest Posts
-
According To Researchers Most People Listen At What Proficiency
Apr 21, 2025
-
Rn Foundational Concepts Of Mental Health Nursing Assessment
Apr 21, 2025
-
Net Exports Of A Country Are The Value Of
Apr 21, 2025
-
Periodic Table Of Elements Worksheet Answer Key
Apr 21, 2025
-
Letrs Unit 1 Session 3 Check For Understanding Answers
Apr 21, 2025
Related Post
Thank you for visiting our website which covers about What Is The Shape Of The Histogram Below . We hope the information provided has been useful to you. Feel free to contact us if you have any questions or need further assistance. See you next time and don't miss to bookmark.