What Is A Major Weakness Of Observational Studies
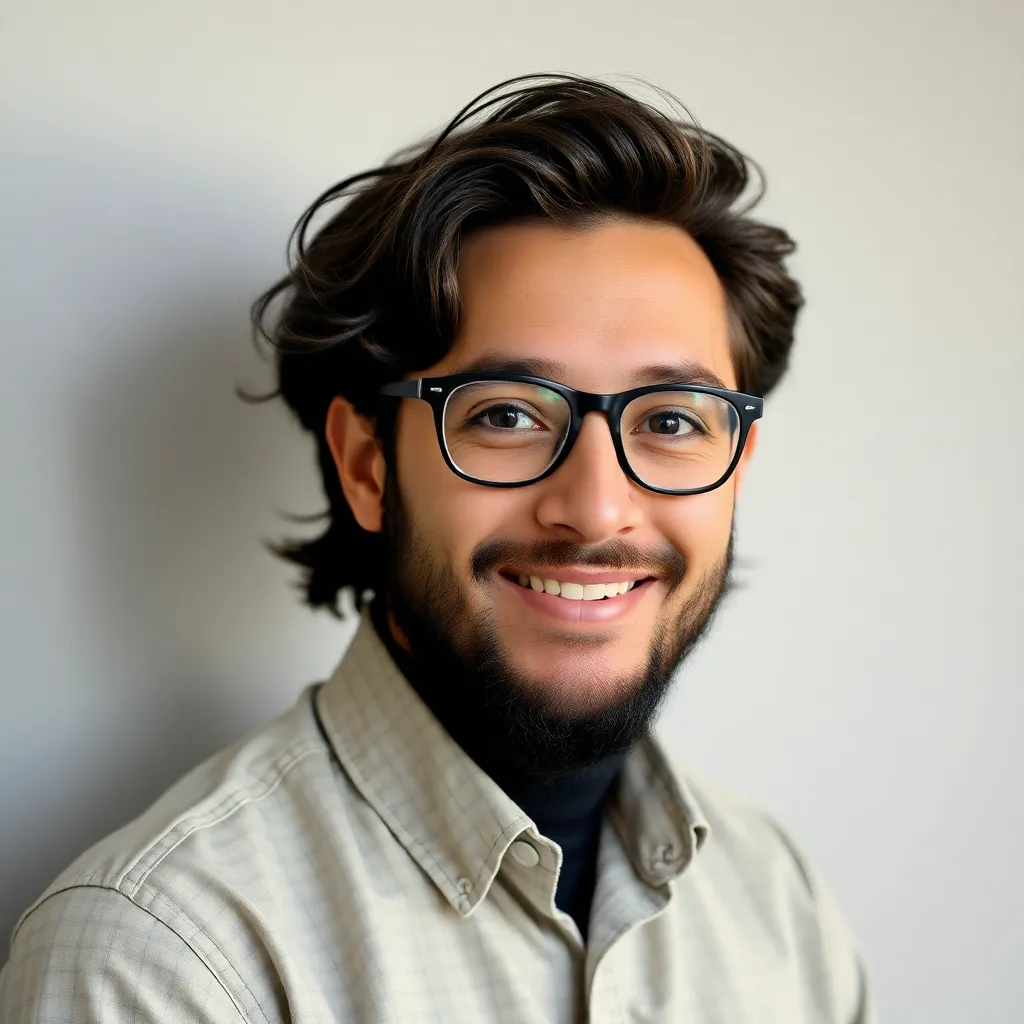
New Snow
Apr 24, 2025 · 6 min read

Table of Contents
What is a Major Weakness of Observational Studies? The Limitations of Correlation and Causation
Observational studies, a cornerstone of epidemiological research and many other scientific fields, offer invaluable insights into the relationships between variables. They allow researchers to examine phenomena in their natural settings, avoiding the ethical and practical challenges of manipulating variables directly, as in experimental studies. However, a major weakness of observational studies lies in their inherent inability to definitively establish causation. While they can identify correlations, they struggle to disentangle the complex web of factors that might contribute to an observed association, leading to potential biases and misinterpretations. This article will delve deep into this fundamental limitation, exploring various types of biases and the challenges they pose to drawing reliable conclusions from observational data.
The Correlation-Causation Fallacy: The Heart of the Weakness
The most significant weakness of observational studies is their susceptibility to the correlation-causation fallacy. This fallacy arises when a researcher observes a correlation between two variables and incorrectly concludes that one variable causes the other. Correlation simply indicates an association; it doesn't imply causation. Many factors can contribute to an observed correlation, including:
1. Confounding Variables: The Unseen Influencers
Confounding variables are lurking variables that influence both the independent and dependent variables, creating a spurious association. These variables are not directly measured in the study but can significantly distort the relationship under investigation. For example, an observational study might find a correlation between coffee consumption and heart disease. However, smoking could be a confounding variable. Smokers might be more likely to drink coffee and have a higher risk of heart disease. The observed correlation between coffee and heart disease might, therefore, be entirely due to the confounding effect of smoking.
Identifying and controlling for confounding variables is crucial in observational studies. Researchers use various statistical techniques, such as stratification, regression analysis, and propensity score matching, to attempt to minimize the impact of confounding. However, it is impossible to account for all potential confounders, especially those that are unknown or unmeasured. This limitation significantly weakens the ability of observational studies to establish causal relationships.
2. Reverse Causality: The Chicken-and-Egg Problem
Another significant challenge is reverse causality. This occurs when the direction of the causal relationship is reversed from what is initially hypothesized. For example, an observational study might find a correlation between stress levels and the development of depression. However, it's possible that depression itself leads to increased stress, rather than stress causing depression. The directionality of the causal link is not clear from the observational data alone. This ambiguity undermines the ability to make confident causal inferences.
3. Selection Bias: A Skewed Perspective
Selection bias arises when the participants in the study are not representative of the broader population of interest. This bias can occur during the recruitment process, leading to a sample that systematically differs from the population in terms of relevant characteristics. For example, if a study on the effects of exercise on weight loss only recruits participants who are already motivated to exercise, the results might overestimate the effectiveness of exercise in promoting weight loss. The sample is not representative of the general population, which includes people with varying levels of motivation and commitment to exercise.
Several types of selection bias can impact observational studies:
- Sampling bias: This occurs when the sample is not representative of the target population.
- Volunteer bias: Participants who volunteer for a study may differ systematically from those who do not.
- Attrition bias: Bias introduced when participants drop out of the study, potentially leaving a skewed sample.
Addressing selection bias requires careful consideration of sampling methods, recruitment strategies, and strategies to minimize participant attrition. Robust statistical analyses can sometimes mitigate the impact of selection bias, but it often remains a significant limitation.
4. Information Bias: Inaccurate Data Collection
Information bias arises from systematic errors in the collection or measurement of data. This can occur due to various reasons, including:
- Recall bias: Participants may inaccurately recall past events or exposures, leading to biased responses. This is especially relevant in retrospective studies where researchers rely on participants' memories.
- Interviewer bias: The interviewer's preconceived notions or biases can influence how questions are asked and how responses are recorded.
- Measurement bias: Inaccurate or unreliable instruments or methods used to collect data can introduce systematic errors.
Minimizing information bias necessitates rigorous data collection protocols, well-trained interviewers, validated measurement tools, and blind or double-blind study designs whenever feasible.
5. Publication Bias: A Skewed Landscape of Research
Publication bias is a systemic problem in scientific research where studies with positive or statistically significant results are more likely to be published than those with negative or null findings. This bias can skew the overall understanding of a phenomenon, as the published literature might overrepresent the strength of an association. This impacts observational studies as their results are subjected to the same publishing pressures as other types of studies.
Mitigating the Weaknesses: Strategies for Stronger Observational Studies
While observational studies cannot definitively prove causation, researchers can employ various strategies to strengthen their validity and minimize the impact of biases:
- Rigorous Study Design: A carefully designed study with clear inclusion and exclusion criteria, detailed data collection protocols, and a large, representative sample can enhance the reliability of the findings.
- Advanced Statistical Techniques: Statistical methods such as propensity score matching, inverse probability weighting, and instrumental variables can help to adjust for confounding and improve causal inference.
- Multiple Studies and Meta-analysis: Combining the results of multiple observational studies through meta-analysis can increase the statistical power and reduce the influence of individual study biases.
- Triangulation with Experimental Evidence: Whenever possible, combining observational findings with results from randomized controlled trials can strengthen causal inferences. Observational studies can generate hypotheses, and experimental studies can then rigorously test those hypotheses.
- Sensitivity Analyses: Assessing the impact of potential biases on the results through sensitivity analyses can provide a more nuanced understanding of the study's limitations.
Conclusion: The Value and Limitations of Observational Studies
Observational studies are powerful tools for generating hypotheses, exploring complex relationships, and providing insights into real-world phenomena. However, their inability to definitively establish causation is a major limitation. Researchers must carefully consider the potential for confounding, bias, and other limitations when interpreting the results of observational studies. Transparency about these limitations is crucial for responsible scientific communication. By acknowledging these weaknesses and employing appropriate methodological strategies, researchers can maximize the value of observational studies while avoiding misleading conclusions about causality. While observational studies cannot replace randomized controlled trials for definitively establishing causal links, their value in generating hypotheses, exploring complex relationships, and informing public health interventions remains undeniable. A nuanced understanding of their strengths and weaknesses is essential for interpreting their results responsibly and accurately.
Latest Posts
Latest Posts
-
Drag Each Label To The Appropriate Target
Apr 24, 2025
-
Ati Comprehensive Physical Assessment Of A Child
Apr 24, 2025
-
The Optic Disc Is Known As The Blind Spot Because
Apr 24, 2025
-
A Researcher Wants To Do A Web Based Survey
Apr 24, 2025
-
Hidden Figures Movie Guide Answer Key
Apr 24, 2025
Related Post
Thank you for visiting our website which covers about What Is A Major Weakness Of Observational Studies . We hope the information provided has been useful to you. Feel free to contact us if you have any questions or need further assistance. See you next time and don't miss to bookmark.