Use Of Simple Linear Regression Analysis Assumes That
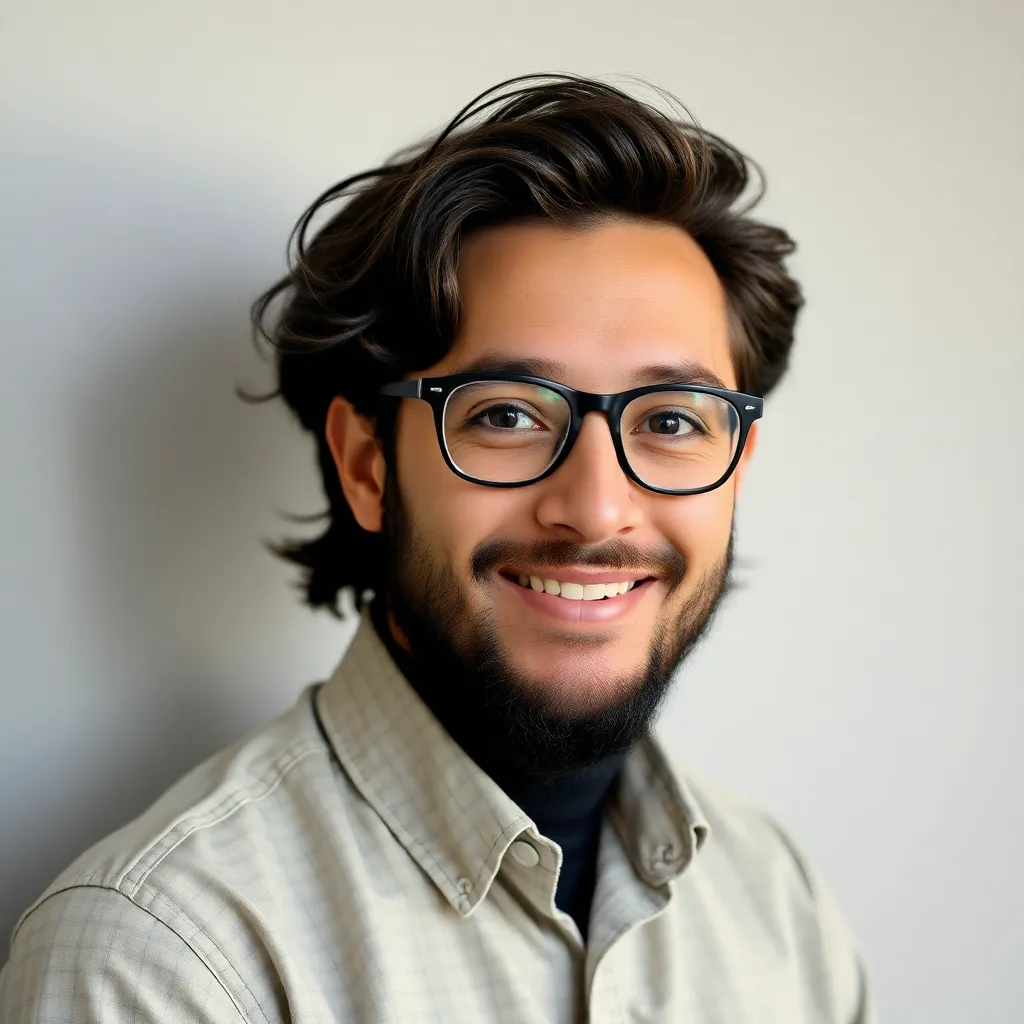
New Snow
Apr 26, 2025 · 6 min read

Table of Contents
Assumptions of Simple Linear Regression Analysis: A Comprehensive Guide
Simple linear regression is a powerful statistical tool used to model the relationship between two continuous variables: a dependent variable (Y) and an independent variable (X). However, the accuracy and reliability of the results depend heavily on several key assumptions being met. Violating these assumptions can lead to inaccurate predictions, misleading interpretations, and unreliable statistical inferences. This article will delve deep into the assumptions of simple linear regression analysis, explaining each one in detail, exploring the consequences of their violation, and suggesting methods for assessing and addressing them.
The Core Assumptions of Simple Linear Regression
The validity of simple linear regression hinges on several crucial assumptions. These assumptions relate to the relationship between the independent and dependent variables, the distribution of the residuals (the errors in the model's predictions), and the overall data structure. Let's explore each assumption meticulously:
1. Linearity
This is arguably the most fundamental assumption. Linearity assumes a linear relationship exists between the independent variable (X) and the dependent variable (Y). This means that a change in X is associated with a proportional change in Y. The relationship can be represented by a straight line.
Consequences of Violation: If the relationship is non-linear, the regression line will poorly fit the data, leading to biased and inefficient estimates of the regression coefficients. The model will fail to accurately capture the true relationship between X and Y.
Assessment: Visual inspection of a scatter plot of X and Y is the simplest method. A clear, straight-line pattern supports linearity. However, more rigorous methods include residual plots (discussed later). Non-linear relationships might be addressed by transforming the variables (e.g., logarithmic, square root transformations) or using non-linear regression models.
2. Independence of Errors
The independence of errors assumption states that the residuals (the differences between the observed Y values and the values predicted by the model) are independent of each other. This means that the error associated with one observation doesn't influence the error associated with another observation. This assumption is particularly crucial when dealing with time-series data where consecutive observations might be correlated.
Consequences of Violation: Violation of this assumption leads to underestimated standard errors, resulting in inflated t-statistics and potentially incorrect conclusions about the significance of the regression coefficients. This is particularly problematic in time-series data where autocorrelation (correlation between consecutive errors) can significantly bias results.
Assessment: The Durbin-Watson test is commonly used to detect autocorrelation. Values close to 2 suggest no autocorrelation. Graphical methods, such as plotting the residuals against time (for time-series data) or against predicted values, can also reveal patterns suggesting dependence.
3. Homoscedasticity (Constant Variance of Errors)
Homoscedasticity implies that the variance of the errors is constant across all levels of the independent variable. In other words, the spread of the residuals should be roughly the same for all values of X. The opposite, where the variance of errors changes across the range of X, is called heteroscedasticity.
Consequences of Violation: Heteroscedasticity leads to inefficient and potentially biased estimates of the regression coefficients. Standard errors are often underestimated, leading to inflated t-statistics and an increased risk of Type I errors (rejecting a true null hypothesis).
Assessment: Residual plots are crucial here. Plotting residuals against predicted values or the independent variable should show a random scatter of points with roughly constant vertical spread. Funnel-shaped patterns indicate heteroscedasticity. Weighted least squares regression can be used to address heteroscedasticity.
4. Normality of Errors
The normality of errors assumption posits that the residuals are normally distributed. This means the distribution of the errors should be approximately bell-shaped, symmetrical around a mean of zero. This assumption is particularly important for making inferences about the population parameters (e.g., confidence intervals and hypothesis tests).
Consequences of Violation: While simple linear regression is relatively robust to violations of normality, especially with larger sample sizes, severe departures from normality can affect the accuracy of p-values and confidence intervals, particularly when the sample size is small. This can lead to incorrect inferences about the significance of the regression coefficients.
Assessment: Histograms, Q-Q plots (quantile-quantile plots), and normality tests (e.g., Shapiro-Wilk test, Kolmogorov-Smirnov test) can assess the normality of residuals. Q-Q plots graphically compare the quantiles of the residuals to the quantiles of a normal distribution. Significant departures from a straight line suggest non-normality.
5. No Multicollinearity (Simple Linear Regression Specific)
This assumption is unique to simple linear regression because it only involves one independent variable. Multicollinearity refers to the correlation between predictor variables. In simple linear regression, this is not a concern since only one predictor exists. However, it becomes critically important in multiple linear regression, where multiple independent variables are involved. High multicollinearity between predictors can inflate the variance of the regression coefficients, making it difficult to determine their individual effects.
Consequences of Violation (In Multiple Linear Regression): Unstable and unreliable coefficient estimates. It makes it difficult to interpret the individual effects of the predictors.
Assessment (In Multiple Linear Regression): Variance Inflation Factor (VIF) is a common diagnostic tool. VIF values greater than 5 or 10 generally indicate problematic multicollinearity.
Dealing with Assumption Violations
When assumptions are violated, several strategies can be employed to mitigate the impact:
-
Data Transformation: Transforming the dependent and/or independent variables (e.g., logarithmic, square root, reciprocal transformations) can often address non-linearity and heteroscedasticity.
-
Weighted Least Squares Regression: This technique assigns different weights to observations, giving more weight to those with smaller variances. This can address heteroscedasticity.
-
Robust Regression Methods: These methods are less sensitive to outliers and violations of normality assumptions.
-
Non-parametric Methods: If assumptions are severely violated, non-parametric methods, which do not rely on distributional assumptions, might be more appropriate.
-
Increasing Sample Size: A larger sample size can sometimes mitigate the impact of violations of normality and homoscedasticity.
Conclusion: The Importance of Assumption Checking
Understanding and checking the assumptions of simple linear regression is crucial for obtaining reliable and meaningful results. Ignoring these assumptions can lead to inaccurate predictions, misleading interpretations, and flawed conclusions. By carefully assessing these assumptions and employing appropriate techniques to address violations, researchers can ensure the validity and robustness of their regression models. Remember, the goal is not necessarily to perfectly satisfy every assumption but to understand their implications and take appropriate steps to minimize their impact on the analysis. A thorough understanding of these assumptions and their implications is essential for anyone working with simple linear regression analysis. Always remember to visualize your data, examine residual plots, and consider the context of your data when interpreting your results.
Latest Posts
Latest Posts
-
What Impact Has Machine Learning Made On The Marketing Industry
Apr 27, 2025
-
Which Three Statements Characterize Udp Choose Three
Apr 27, 2025
-
A Negative Externality Or Additional Social Cost Occurs When
Apr 27, 2025
-
Nursing Student Printable Drug Card Template
Apr 27, 2025
-
An Article Reports That Blue Eyed People Earn Less
Apr 27, 2025
Related Post
Thank you for visiting our website which covers about Use Of Simple Linear Regression Analysis Assumes That . We hope the information provided has been useful to you. Feel free to contact us if you have any questions or need further assistance. See you next time and don't miss to bookmark.