The Probability Distribution Of Is Called A Distribution.
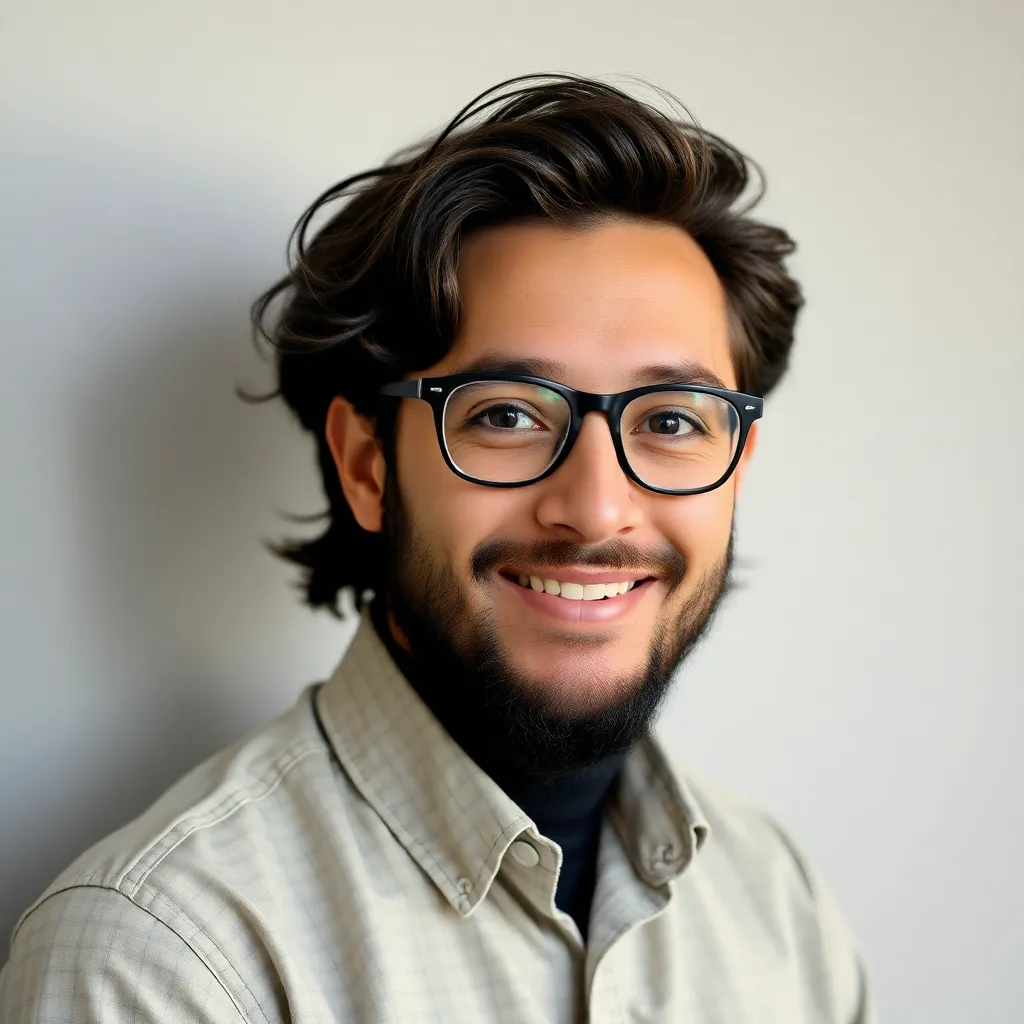
New Snow
Apr 21, 2025 · 6 min read

Table of Contents
The Probability Distribution Called a Distribution: A Comprehensive Guide
The question "The probability distribution of is called a distribution" is incomplete. A probability distribution isn't simply "called a distribution." It's named after the specific characteristics of the data it describes. To understand this, we need to explore various probability distributions and the conditions under which they arise. This comprehensive guide delves into numerous common distributions, highlighting their defining features and applications.
Understanding Probability Distributions
Before diving into specific distributions, let's establish a foundational understanding. A probability distribution is a mathematical function that provides the probabilities of occurrence of different possible outcomes for a random variable. It's a complete description of the possible values a random variable can take and their associated probabilities. These distributions can be discrete (for variables that can only take on specific, separate values) or continuous (for variables that can take on any value within a given range).
Key Characteristics of Probability Distributions
Several key characteristics help us understand and compare different probability distributions:
- Mean (Expected Value): The average value of the random variable.
- Variance: A measure of the spread or dispersion of the data around the mean. The square root of the variance is the standard deviation.
- Skewness: A measure of the asymmetry of the distribution. A positive skew indicates a longer tail to the right, while a negative skew indicates a longer tail to the left.
- Kurtosis: A measure of the "tailedness" of the probability distribution. High kurtosis indicates heavy tails and sharp peak, while low kurtosis indicates light tails and a flat top.
Common Probability Distributions
Let's explore some of the most frequently encountered probability distributions:
1. Normal Distribution (Gaussian Distribution)
Arguably the most important distribution in statistics, the normal distribution is characterized by its bell-shaped curve. It's symmetrical around its mean, and its parameters are the mean (µ) and standard deviation (σ).
Characteristics:
- Symmetrical: The mean, median, and mode are all equal.
- Bell-shaped: The probability density function is a smooth, continuous curve.
- Defined by mean (µ) and standard deviation (σ): These parameters completely determine the shape and location of the distribution.
Applications:
The normal distribution is ubiquitous in numerous fields, including:
- Natural phenomena: Heights, weights, and blood pressure often follow a normal distribution.
- Statistical inference: Many statistical tests assume that the data is normally distributed.
- Finance: Modeling asset returns.
- Engineering: Quality control and process optimization.
2. Binomial Distribution
The binomial distribution models the probability of obtaining a certain number of successes in a fixed number of independent Bernoulli trials (trials with only two possible outcomes: success or failure).
Characteristics:
- Discrete: The random variable can only take on integer values (0, 1, 2, ..., n).
- Two parameters: n (number of trials) and p (probability of success).
- Independent trials: The outcome of one trial does not affect the outcome of another.
Applications:
- Quality control: Determining the probability of a certain number of defective items in a batch.
- Medical research: Assessing the effectiveness of a treatment based on the number of successful outcomes.
- Polling: Estimating the proportion of people who support a particular candidate.
3. Poisson Distribution
The Poisson distribution describes the probability of a given number of events occurring in a fixed interval of time or space if these events occur with a known average rate and independently of the time since the last event.
Characteristics:
- Discrete: The random variable can only take on non-negative integer values (0, 1, 2, ...).
- One parameter: λ (the average rate of events).
- Events are independent: The occurrence of one event does not affect the probability of another event occurring.
Applications:
- Customer arrival: Modeling the number of customers arriving at a store in an hour.
- Accident rates: Predicting the number of accidents on a particular highway section.
- Defect analysis: Determining the number of defects per unit in a manufacturing process.
4. Uniform Distribution
The uniform distribution assigns equal probability to all values within a specified range.
Characteristics:
- Continuous (or discrete): Can be either, depending on the specific context.
- Two parameters: a (minimum value) and b (maximum value).
- Constant probability density: The probability of observing any value within the range [a, b] is the same.
Applications:
- Simulation: Generating random numbers within a specific interval.
- Random sampling: Selecting a random value from a set of possibilities.
5. Exponential Distribution
The exponential distribution describes the time until an event occurs in a Poisson process.
Characteristics:
- Continuous: The random variable can take on any non-negative value.
- One parameter: λ (the rate parameter, which is the reciprocal of the mean).
- Memoryless property: The probability of the event occurring in the future is independent of how long it has already been waiting.
Applications:
- Reliability analysis: Modeling the lifespan of components or systems.
- Queuing theory: Analyzing waiting times in queues.
- Survival analysis: Studying the time until an event, such as death or failure.
6. Gamma Distribution
The gamma distribution is a flexible distribution that can model a wide range of shapes. It is often used to model waiting times or durations.
Characteristics:
- Continuous: The random variable can take on any positive value.
- Two parameters: shape (k) and scale (θ). The shape parameter determines the skewness, while the scale parameter affects the spread.
Applications:
- Modeling waiting times: Similar to exponential distribution but more flexible due to two parameters.
- Image processing: Modeling pixel intensities.
- Financial modeling: Modeling the time until default.
7. Beta Distribution
The beta distribution is defined on the interval [0, 1] and is useful for modeling probabilities or proportions.
Characteristics:
- Continuous: The random variable is confined to the range [0,1].
- Two shape parameters: α and β. These parameters control the shape of the distribution.
Applications:
- Bayesian statistics: Representing prior distributions for probabilities.
- Modeling proportions: Estimating the probability of success in a binomial experiment.
8. Chi-Square Distribution
The chi-square distribution is used extensively in hypothesis testing and is related to the normal distribution.
Characteristics:
- Continuous: The random variable takes on non-negative values.
- One parameter: Degrees of freedom (k), which determines the shape of the distribution.
Applications:
- Goodness-of-fit tests: Assessing how well a sample data fits a theoretical distribution.
- Tests of independence: Determining whether two categorical variables are independent.
9. t-Distribution
The t-distribution is similar to the normal distribution but has heavier tails, especially for small sample sizes. It's crucial in statistical inference when the population standard deviation is unknown.
Characteristics:
- Continuous: The random variable can take on any real value.
- One parameter: Degrees of freedom (k), influencing the shape.
Applications:
- Hypothesis testing: When the population standard deviation is unknown.
- Confidence intervals: Constructing confidence intervals for the mean.
10. F-Distribution
The F-distribution is used in ANOVA (analysis of variance) and other statistical tests comparing variances of two or more groups.
Characteristics:
- Continuous: The random variable takes on only positive values.
- Two parameters: Degrees of freedom for the numerator (k1) and the denominator (k2).
Applications:
- ANOVA: Testing for differences in means between multiple groups.
- Regression analysis: Testing the significance of regression models.
Conclusion
This guide provides an overview of several common probability distributions. Each distribution has unique characteristics that make it suitable for modeling specific types of data and answering particular statistical questions. Understanding these distributions is fundamental to many areas of statistics, data analysis, and decision-making. Remember that the "distribution" referred to in the original incomplete question depends entirely on the context and the specific properties of the data being analyzed. Choosing the right distribution is crucial for accurate and meaningful analysis. Further research into specific applications and advanced concepts related to each distribution is encouraged for a deeper understanding.
Latest Posts
Latest Posts
-
Fixed Annuities Provide Each Of The Following Except
Apr 21, 2025
-
The Facial Gains Guide Pdf Free Download
Apr 21, 2025
-
Relative Highness Or Lowness Of A Sound
Apr 21, 2025
-
Common Aseptic Transfers And Inoculation Methods Data Sheet 1 4
Apr 21, 2025
-
The Cross Sectional Approach To Developmental Research Compares
Apr 21, 2025
Related Post
Thank you for visiting our website which covers about The Probability Distribution Of Is Called A Distribution. . We hope the information provided has been useful to you. Feel free to contact us if you have any questions or need further assistance. See you next time and don't miss to bookmark.