Sampling Is Preferred When The Objective Is To Increase Precision
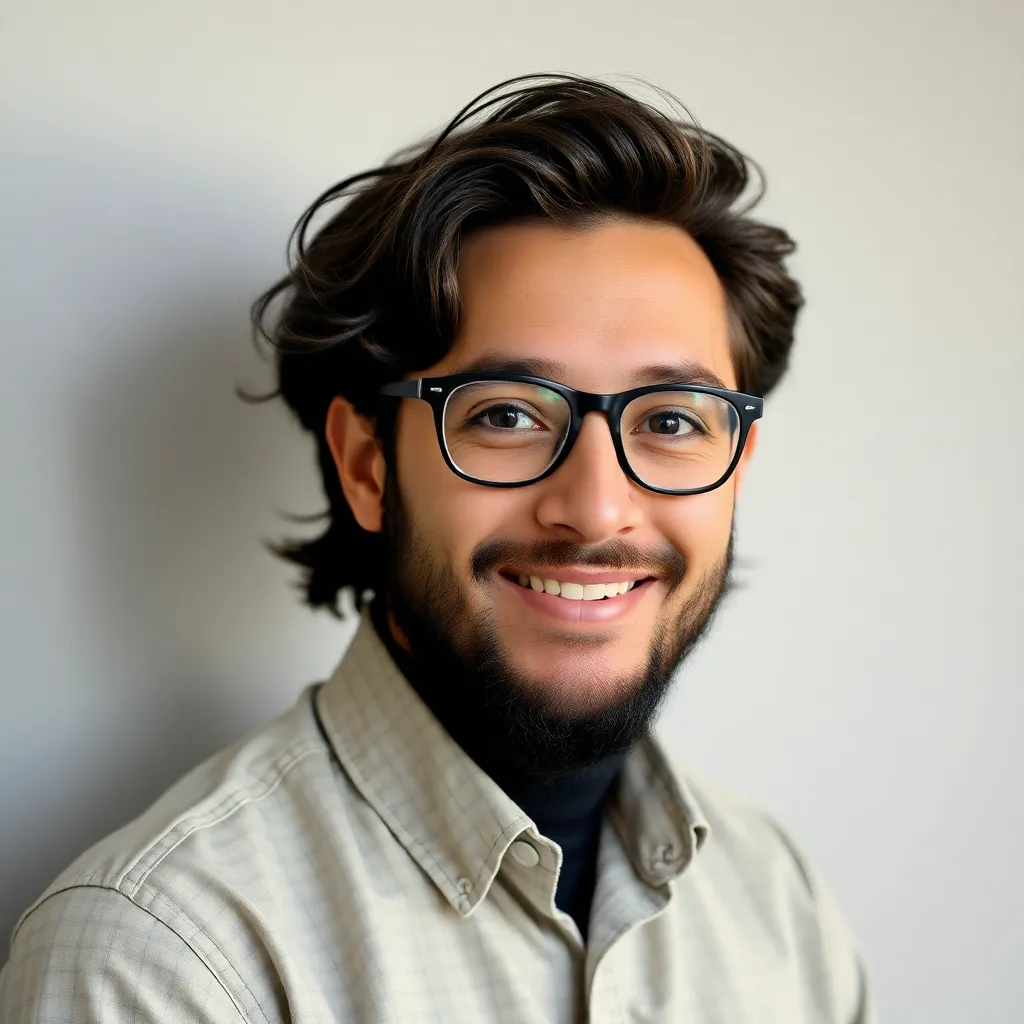
New Snow
Apr 21, 2025 · 6 min read

Table of Contents
Sampling is Preferred When the Objective is to Increase Precision
Sampling, the process of selecting a subset of individuals from a larger population, is a cornerstone of various fields, from statistics and research to quality control and market analysis. While studying an entire population (a census) might seem ideal, it's often impractical, expensive, and even impossible. This is where the strategic power of sampling shines, especially when the primary goal is to enhance precision. This article delves deep into why sampling is the preferred approach when high precision is the objective, exploring its advantages, various sampling techniques, and considerations for maximizing precision in your chosen method.
Why Sampling Beats a Census for Precision
The pursuit of precision in data analysis often clashes with the limitations of complete data collection. Let's examine why sampling surpasses a census in achieving this goal:
1. Cost-Effectiveness:
Conducting a census requires substantial resources. The cost associated with data collection, processing, and analysis escalates dramatically with population size. Sampling significantly reduces these costs by focusing on a smaller, manageable group, allowing for more efficient resource allocation. This freed-up budget can be reinvested in improving the quality of the sampling process itself, thereby leading to higher precision.
2. Time Efficiency:
Time is a critical factor in many research and analytical endeavors. A census takes considerably longer to complete than a sample survey. The shorter timeframe afforded by sampling allows for quicker results, enabling faster decision-making and responses to evolving situations. This timely information, even if based on a sample, can be significantly more precise and valuable than delayed information derived from a complete census.
3. Reduced Error:
Paradoxically, aiming for complete data collection can introduce more errors. The sheer volume of data in a census increases the likelihood of human error during data collection, processing, and analysis. Sampling allows for stricter quality control and a more focused approach, mitigating the accumulation of errors inherent in large-scale data collection. This control contributes directly to increased precision.
4. Accessibility:
Certain populations are difficult, if not impossible, to access completely. Think about populations spread across vast geographical areas, rare species in remote locations, or elusive online communities. Sampling allows researchers to access these otherwise inaccessible populations, enabling data collection that would be otherwise unattainable, potentially resulting in more precise insights.
5. Detailed Analysis:
Ironically, the massive dataset generated by a census can hinder detailed analysis. Sifting through an immense amount of information can be overwhelming, leading to superficial analysis and less precise conclusions. A well-designed sample allows for a more in-depth examination of the data, revealing subtle trends and patterns that may be obscured in a census.
Sampling Techniques for Precision Enhancement
Several sampling techniques cater to different research objectives and population characteristics. The choice of technique significantly impacts the precision of the results. Here are some prominent methods:
1. Simple Random Sampling:
This technique involves selecting sample units purely by chance, ensuring each member of the population has an equal probability of being chosen. While straightforward, it might not always be the most efficient for achieving high precision, especially with a heterogeneous population. However, its simplicity makes it a great baseline for comparison with other techniques.
2. Stratified Sampling:
Stratified sampling divides the population into homogeneous subgroups (strata) based on relevant characteristics (e.g., age, gender, income). Then, a random sample is drawn from each stratum. This technique enhances precision by ensuring representation from all segments of the population, reducing sampling error and providing a more accurate reflection of the overall population.
3. Cluster Sampling:
Cluster sampling divides the population into clusters, typically geographical areas or organizational units. A random sample of clusters is selected, and all units within the selected clusters are included in the sample. While potentially less precise than stratified sampling if clusters aren't homogenous, it's more cost-effective for geographically dispersed populations.
4. Systematic Sampling:
In systematic sampling, every kth unit from the population is selected after a random starting point. This is a simple and efficient method, but it's crucial to ensure that the population isn't cyclic or patterned to avoid bias. This technique offers a balance between simplicity and potentially high precision.
5. Multistage Sampling:
Multistage sampling combines various sampling techniques in multiple stages. For example, it might start with cluster sampling to select regions and then use stratified sampling within those regions to ensure representation across different subgroups. This complex method is particularly useful for large and diverse populations, allowing for increased precision by incorporating multiple levels of stratification and control.
6. Quota Sampling:
Quota sampling, a non-probability method, ensures that the sample reflects the population's proportions regarding specific characteristics. While useful for ensuring representation, it lacks the randomness of probability sampling and may introduce bias, potentially compromising precision if not carefully executed.
Maximizing Precision in Sampling: Key Considerations
Several factors contribute to achieving high precision in sampling:
1. Sample Size:
The sample size is crucial. Larger samples generally yield more precise results, as they reduce sampling error. However, the relationship is not linear; the gains diminish with increasing sample size beyond a certain point. Appropriate sample size calculation based on desired precision, confidence level, and population variability is essential.
2. Sampling Frame:
The sampling frame is the list from which the sample is drawn. A comprehensive and accurate sampling frame is paramount. Inaccuracies or biases in the sampling frame can lead to significant errors and reduced precision.
3. Data Collection Methods:
The precision of the results is heavily influenced by the data collection methods employed. Clear, concise questionnaires, well-trained interviewers, and robust data entry processes all minimize error and contribute to higher precision.
4. Data Analysis Techniques:
The chosen analytical methods play a significant role. Appropriate statistical techniques should be used to account for sampling variability and estimate the precision of the results. Confidence intervals and margin of error estimations are crucial for accurately communicating the level of precision.
5. Addressing Non-Response:
Non-response, the failure to collect data from some selected units, can introduce bias and reduce precision. Strategies to minimize non-response, such as follow-up attempts and incentives, should be incorporated.
6. Statistical Power:
Statistical power refers to the ability of a study to detect a real effect. Adequate statistical power is necessary to ensure that the sample size is large enough to detect meaningful differences and achieve the desired level of precision. A power analysis can help determine the appropriate sample size to achieve the desired power level.
Conclusion: Precision Through Strategic Sampling
Sampling, when strategically implemented, significantly enhances precision in research and data analysis. It provides a cost-effective, timely, and manageable alternative to exhaustive censuses. The choice of sampling technique, careful consideration of sample size and the sampling frame, rigorous data collection methods, and appropriate statistical analysis are all vital to maximizing precision and drawing accurate conclusions from the sample data. By understanding and employing these principles, researchers and analysts can harness the power of sampling to achieve accurate and insightful results, even when dealing with vast and complex populations. The pursuit of precision doesn't necessitate a complete enumeration; rather, it often flourishes through the intelligent application of well-designed sampling strategies.
Latest Posts
Latest Posts
-
Atoms Elements And Molecules Warm Up Answer Key
Apr 21, 2025
-
Which Of The Following Is An Example Of Secondary Research
Apr 21, 2025
-
The History Of The Atom Worksheet
Apr 21, 2025
-
Which Of The Following Is Equal To The Expression Below
Apr 21, 2025
-
Cells Alive Worksheet Answer Key Pdf
Apr 21, 2025
Related Post
Thank you for visiting our website which covers about Sampling Is Preferred When The Objective Is To Increase Precision . We hope the information provided has been useful to you. Feel free to contact us if you have any questions or need further assistance. See you next time and don't miss to bookmark.