Probabilities That Are Estimated From Observed Frequencies Are Called
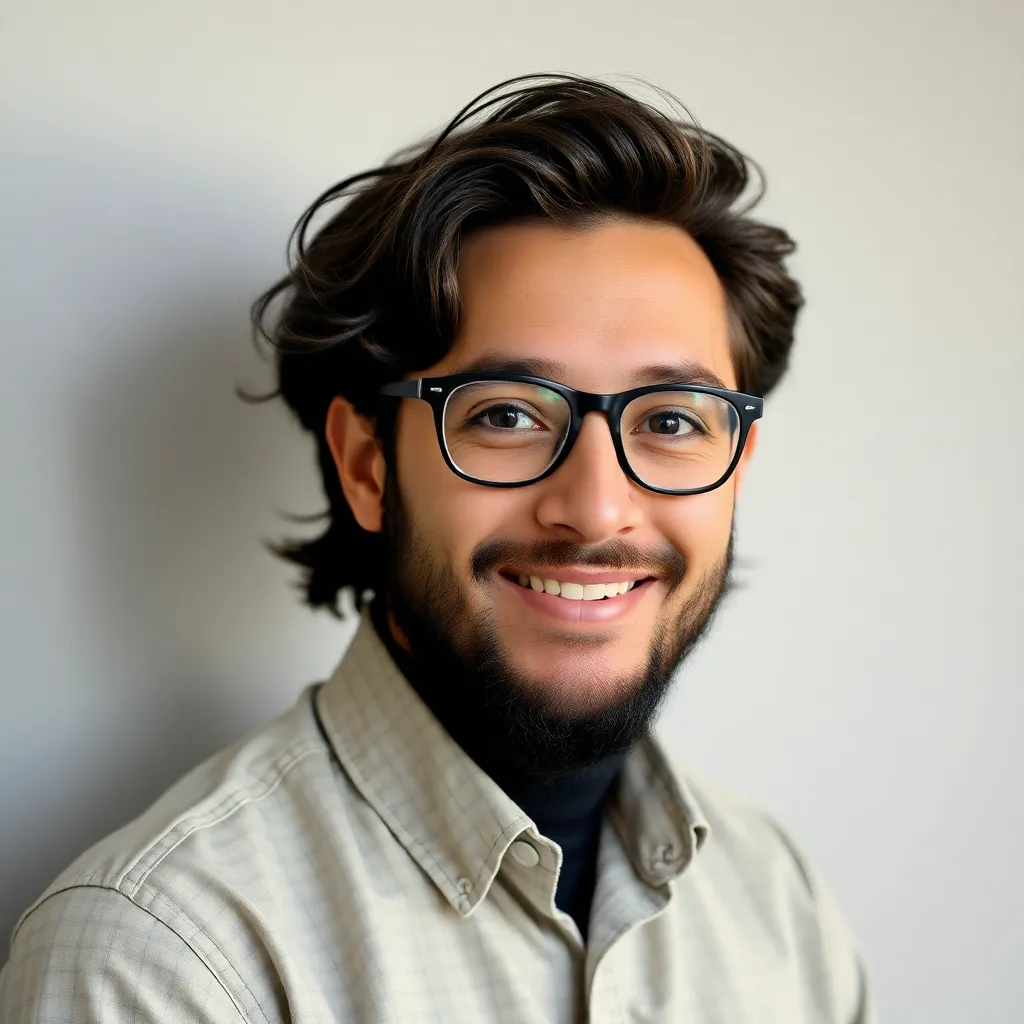
New Snow
Apr 27, 2025 · 7 min read

Table of Contents
Probabilities Estimated from Observed Frequencies: A Deep Dive into Empirical Probability
Probabilities that are estimated from observed frequencies are called empirical probabilities, also known as relative frequencies or experimental probabilities. Unlike theoretical probabilities, which are derived from mathematical models and assumptions, empirical probabilities are based on real-world observations and data collected from experiments or surveys. Understanding the nuances of empirical probability is crucial in various fields, from statistics and data science to risk assessment and decision-making. This article will explore the concept of empirical probability in detail, covering its calculation, applications, limitations, and comparisons with theoretical probability.
Understanding Empirical Probability
Empirical probability focuses on the frequency of an event occurring within a specific sample. It's a practical approach that uses observed data to estimate the likelihood of an event happening in the future. The fundamental formula for calculating empirical probability is:
P(A) = (Number of times event A occurred) / (Total number of trials or observations)
Where:
- P(A) represents the empirical probability of event A.
- (Number of times event A occurred) is the frequency of event A in the observed data.
- (Total number of trials or observations) is the total number of trials or observations in the dataset.
Let's illustrate this with an example:
Suppose we're tossing a coin 100 times. We observe that heads appear 53 times. The empirical probability of getting heads in this experiment is:
P(Heads) = 53 / 100 = 0.53
This means that based on our observations, the probability of getting heads is 0.53. Note that this is an estimate; the true probability might be different. The more trials we conduct, the closer the empirical probability is likely to get to the theoretical probability (which is 0.5 for a fair coin).
The Law of Large Numbers and Empirical Probability
A cornerstone of empirical probability is the Law of Large Numbers. This law states that as the number of trials in an experiment increases, the empirical probability of an event will converge towards its true probability. This means that with a sufficiently large sample size, the observed frequency of an event will become a reliable estimate of its actual probability.
However, it's crucial to understand that the Law of Large Numbers doesn't guarantee that the empirical probability will exactly equal the true probability, even with a large number of trials. It only states that the difference between the two will likely become smaller as the sample size grows.
Example: Estimating the Probability of a Defective Item
Imagine a factory producing light bulbs. To estimate the probability of producing a defective bulb, quality control inspectors randomly sample 1000 bulbs. They find 15 defective bulbs. The empirical probability of a defective bulb is:
P(Defective) = 15 / 1000 = 0.015
This suggests that approximately 1.5% of the bulbs produced are defective. However, this is just an estimate based on a sample of 1000 bulbs. The actual percentage of defective bulbs in the entire factory production might be slightly higher or lower. A larger sample size would lead to a more precise estimate.
Empirical Probability vs. Theoretical Probability
It's important to distinguish between empirical and theoretical probability.
-
Theoretical probability: This is based on logical reasoning and mathematical models. It assumes ideal conditions and perfect knowledge of all possible outcomes. For example, the theoretical probability of rolling a 6 on a fair six-sided die is 1/6.
-
Empirical probability: This is based on real-world observations and data. It doesn't rely on assumptions about ideal conditions. The accuracy of empirical probability depends heavily on the quality and size of the data used.
While both types of probability are valuable, they have different strengths and weaknesses. Theoretical probability provides a benchmark for comparison, but it might not reflect the reality of a specific situation. Empirical probability offers a practical estimate based on actual data but can be subject to sampling errors and biases. Often, both approaches are used together to gain a comprehensive understanding of probability.
Applications of Empirical Probability
Empirical probability finds widespread applications in numerous fields:
1. Risk Assessment:
Insurance companies heavily rely on empirical probabilities to assess risks and set premiums. By analyzing historical data on accidents, claims, and other relevant events, they can estimate the likelihood of future events and price their policies accordingly.
2. Quality Control:
Manufacturers use empirical probability to estimate the defect rate of their products. By sampling products and measuring the frequency of defects, they can identify areas for improvement in their production processes.
3. Medical Research:
In clinical trials, researchers use empirical probability to evaluate the effectiveness of new drugs or treatments. By comparing the outcomes in treatment and control groups, they can estimate the probability of success for the new intervention.
4. Weather Forecasting:
Meteorologists use empirical probabilities to predict weather patterns. They analyze historical weather data to estimate the likelihood of various weather events, such as rainfall, temperature changes, and storms.
5. Actuarial Science:
Actuaries use empirical probability to assess financial risks, particularly in the insurance and pensions industries. They analyze demographic data, mortality rates, and other factors to estimate the likelihood of future payouts and manage financial reserves.
6. Machine Learning:
Empirical probability forms the foundation of many machine learning algorithms. These algorithms learn from data and estimate probabilities to make predictions or classifications. For example, spam filters use empirical probabilities based on the frequency of words and other features in emails to classify them as spam or not spam.
Limitations of Empirical Probability
While empirical probability is a powerful tool, it's important to acknowledge its limitations:
-
Sample Size: The accuracy of empirical probability heavily depends on the sample size. Small samples can lead to unreliable estimates, while larger samples generally produce more accurate results.
-
Sampling Bias: If the sample is not representative of the population, the empirical probability will be biased and inaccurate. For example, if we only survey people in a specific geographic area, our estimate of the national average might be misleading.
-
Randomness: Empirical probability assumes that the observed data is randomly sampled from the population. If there's a systematic pattern or bias in the data collection process, the empirical probability will be affected.
-
Changes Over Time: Empirical probabilities are based on past data. If the underlying conditions change over time, the previously estimated probabilities might no longer be accurate. For instance, a historical probability of car accidents might not reflect current safety standards or driving habits.
-
Rare Events: Estimating the probability of rare events using empirical probability can be challenging. It might require an extremely large sample size to observe enough instances of the rare event to obtain a reliable estimate. For example, estimating the probability of a meteor strike requires a vast dataset over a long period.
Improving the Accuracy of Empirical Probability
Several strategies can be used to improve the accuracy of empirical probabilities:
-
Increase the Sample Size: A larger sample size reduces the impact of random variations and leads to more reliable estimates.
-
Ensure Representative Sampling: Using appropriate sampling techniques to ensure the sample is representative of the population minimizes sampling bias.
-
Employ Statistical Methods: Advanced statistical methods can help to account for sampling errors and other sources of uncertainty.
-
Regularly Update Data: Regularly updating the data used to calculate empirical probabilities helps to account for changes over time.
-
Use Multiple Data Sources: Combining data from multiple sources can provide a more comprehensive and accurate picture.
Conclusion
Empirical probability, derived from observed frequencies, offers a practical and powerful approach to estimating the likelihood of events in real-world situations. Its applications span various fields, from risk assessment to machine learning. However, it's crucial to understand its limitations, including the impact of sample size, sampling bias, and changes over time. By employing appropriate methodologies and acknowledging these limitations, we can leverage the strength of empirical probability to make informed decisions and gain valuable insights from data. Remember that combining empirical observations with theoretical understanding often provides the most robust and comprehensive probabilistic assessments.
Latest Posts
Latest Posts
-
Individuals Differ In Their Willingness To Pay For Air Travel
Apr 27, 2025
-
Ap Gov Unit 3 Progress Check Mcq
Apr 27, 2025
-
What Is Troubling Romeo At The Beginning Of The Play
Apr 27, 2025
-
Which Of The Following Molecules Is Achiral
Apr 27, 2025
-
The Main Therapeutic Goal Of Rebt Is To
Apr 27, 2025
Related Post
Thank you for visiting our website which covers about Probabilities That Are Estimated From Observed Frequencies Are Called . We hope the information provided has been useful to you. Feel free to contact us if you have any questions or need further assistance. See you next time and don't miss to bookmark.