Efficient Portfolios Of N Risky Securities Are Portfolios That:
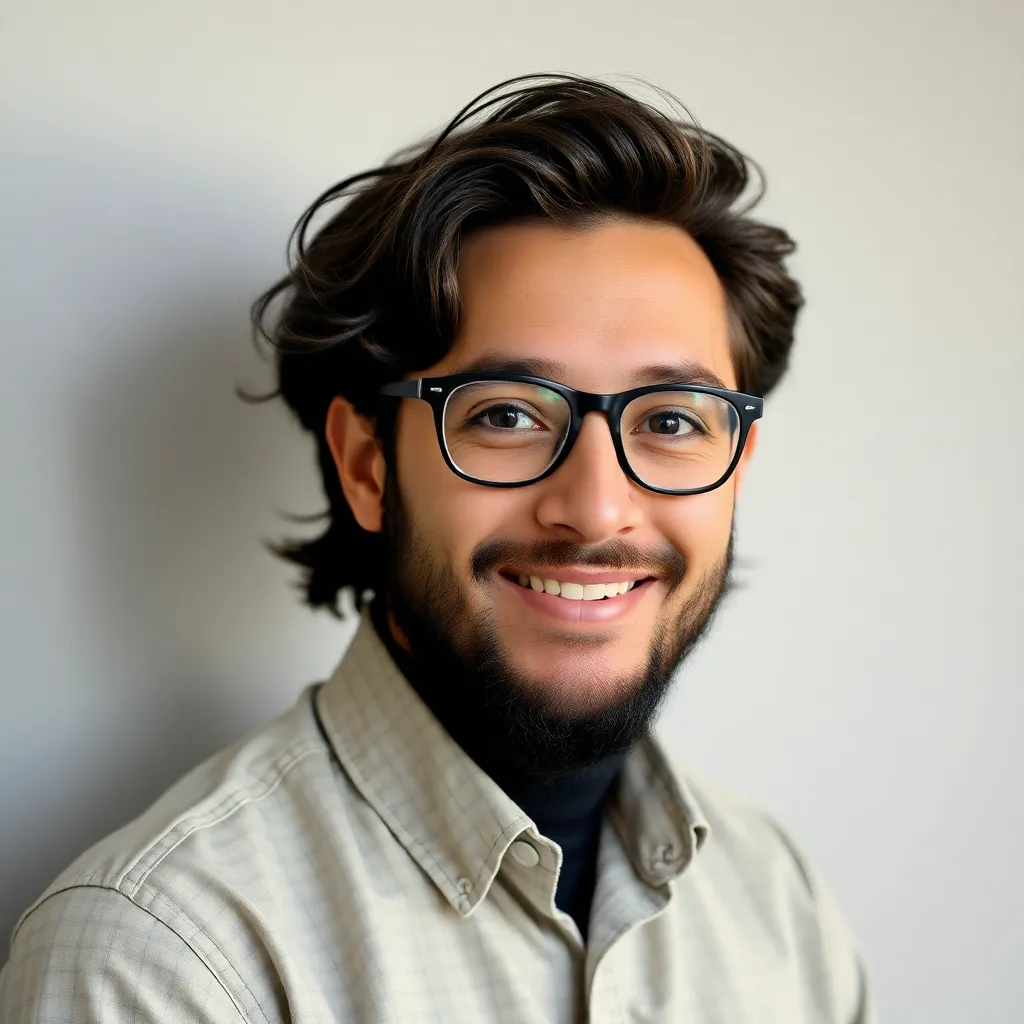
New Snow
Apr 25, 2025 · 6 min read

Table of Contents
Efficient Portfolios of N Risky Securities: A Deep Dive
Efficient portfolios, in the context of modern portfolio theory (MPT), represent the optimal balance between risk and return for a given level of risk aversion. While the concept is often illustrated with two assets, the reality of investment is far more complex, involving numerous securities. Understanding efficient portfolios of n risky securities is crucial for sophisticated investors seeking to optimize their investment strategies. This article will delve into the intricacies of constructing and analyzing these portfolios, exploring the mathematical underpinnings and practical implications.
Understanding the Basics: Risk and Return
Before diving into the complexities of n risky assets, let's establish a foundational understanding of risk and return. In finance, return is the profit or loss on an investment over a specific period, often expressed as a percentage. Risk, on the other hand, is the uncertainty associated with the return. High-risk investments have the potential for high returns but also carry a greater chance of significant losses. Conversely, low-risk investments generally offer lower returns but provide greater stability.
The cornerstone of MPT is the quantification of risk and return. Return is often represented by the expected return (E[R]), which is the weighted average of possible returns, each weighted by its probability. Risk, on the other hand, is typically measured by the standard deviation (σ), or its square, the variance (σ²), which quantifies the dispersion of returns around the expected return. A higher standard deviation indicates greater risk.
The Mean-Variance Framework: A Foundation for Portfolio Optimization
The mean-variance framework, developed by Harry Markowitz, forms the bedrock of modern portfolio theory. This framework posits that investors are risk-averse and aim to maximize their expected return for a given level of risk (or minimize risk for a given level of return). This is achieved by constructing a portfolio that optimally combines different assets.
Within this framework, the expected return of a portfolio (E[Rp]) is a weighted average of the expected returns of individual assets:
E[Rp] = Σ wi * E[Ri]
Where:
E[Rp]
is the expected return of the portfoliowi
is the weight of asset i in the portfolioE[Ri]
is the expected return of asset i
The portfolio's variance (σp²), a measure of its risk, is more complex:
σp² = Σ Σ wi * wj * Cov(Ri, Rj)
Where:
σp²
is the variance of the portfolioCov(Ri, Rj)
is the covariance between the returns of assets i and j.
The covariance term highlights the importance of diversification. If the returns of two assets are negatively correlated (Cov(Ri, Rj) < 0), combining them in a portfolio can reduce the overall risk.
Efficient Frontier: The Set of Optimal Portfolios
The efficient frontier is the graphical representation of all efficient portfolios. An efficient portfolio is one that offers the highest expected return for a given level of risk, or the lowest risk for a given level of expected return. Portfolios that lie below the efficient frontier are dominated because they offer lower returns for the same level of risk or higher risk for the same level of return. Investors should only consider portfolios that lie on the efficient frontier.
Constructing the efficient frontier for n risky assets requires solving a complex optimization problem, typically using numerical methods. This involves finding the weights (wi) that maximize the expected return for a given level of risk (or minimize risk for a given level of return), subject to the constraint that the weights sum to one (Σ wi = 1).
The Role of Covariance Matrix
The covariance matrix is central to portfolio optimization. It's an n x n matrix where each element (i,j) represents the covariance between the returns of asset i and asset j. The diagonal elements represent the variances of individual assets. Accurate estimation of the covariance matrix is crucial for constructing efficient portfolios. However, estimating the covariance matrix accurately, particularly with a large number of assets, presents significant challenges. The accuracy of the covariance matrix directly impacts the accuracy of the resulting efficient frontier.
Incorporating the Risk-Free Asset: The Capital Allocation Line (CAL)
The efficient frontier is further enhanced by incorporating a risk-free asset, such as a government bond. This introduces the Capital Allocation Line (CAL). The CAL represents the set of portfolios formed by combining the risk-free asset with a portfolio of risky assets (often the tangency portfolio). The tangency portfolio is the point on the efficient frontier that has the highest Sharpe ratio. The Sharpe ratio measures the risk-adjusted return, which is the excess return (over the risk-free rate) per unit of risk.
Practical Challenges and Considerations
While the theoretical framework of MPT is elegant, practical application presents several challenges:
- Estimating Expected Returns and Covariance Matrix: Accurately estimating future returns and the covariance matrix is notoriously difficult. Historical data may not be a reliable predictor of future performance, and estimation errors can significantly impact portfolio construction.
- Transaction Costs and Taxes: MPT often ignores transaction costs and taxes, which can significantly erode portfolio returns.
- Non-Normality of Returns: MPT assumes that asset returns are normally distributed. However, this assumption may not hold true in reality, particularly during periods of market stress. Non-normality can lead to higher kurtosis (fat tails) and skewness, which MPT does not fully capture.
- Estimation Risk: The uncertainty associated with estimating inputs (like expected returns and covariances) adds another layer of complexity. This estimation risk should be taken into account during portfolio construction.
Advanced Techniques and Extensions
Several advanced techniques aim to address the limitations of traditional MPT:
- Robust Optimization: Robust optimization techniques aim to construct portfolios that are less sensitive to estimation errors in the input parameters.
- Black-Litterman Model: The Black-Litterman model incorporates investor views into the portfolio optimization process, allowing for a more personalized approach.
- Factor Models: Factor models, such as the Fama-French three-factor model, offer a more sophisticated approach to modeling asset returns by considering various market factors.
- Bayesian Methods: Bayesian methods incorporate prior beliefs about asset returns and covariances, providing a more flexible framework for portfolio optimization.
Conclusion
Constructing efficient portfolios of n risky securities is a complex endeavor requiring a deep understanding of modern portfolio theory, statistical methods, and practical considerations. While the theoretical framework provides a powerful tool for optimizing investment strategies, practical challenges related to parameter estimation, transaction costs, and distributional assumptions need careful consideration. By employing advanced techniques and incorporating a nuanced understanding of market dynamics, investors can strive to build portfolios that balance risk and return effectively, achieving their financial goals. The journey toward efficient portfolio construction is ongoing, constantly evolving with advancements in financial theory and computational capabilities. The key lies in a continuous learning process and adaptation to the ever-changing market landscape.
Latest Posts
Latest Posts
-
6 4 1 Packet Tracer Implement Etherchannel
Apr 25, 2025
-
According To A Recent Survey 47 Percent
Apr 25, 2025
-
The Party By Pam Munoz Ryan
Apr 25, 2025
-
Which Of The Following Cells Transport Sugars Over Long Distances
Apr 25, 2025
-
Which Of The Following Multiple Intelligences Best Describes An Athlete
Apr 25, 2025
Related Post
Thank you for visiting our website which covers about Efficient Portfolios Of N Risky Securities Are Portfolios That: . We hope the information provided has been useful to you. Feel free to contact us if you have any questions or need further assistance. See you next time and don't miss to bookmark.