As The Sample Size Increases The
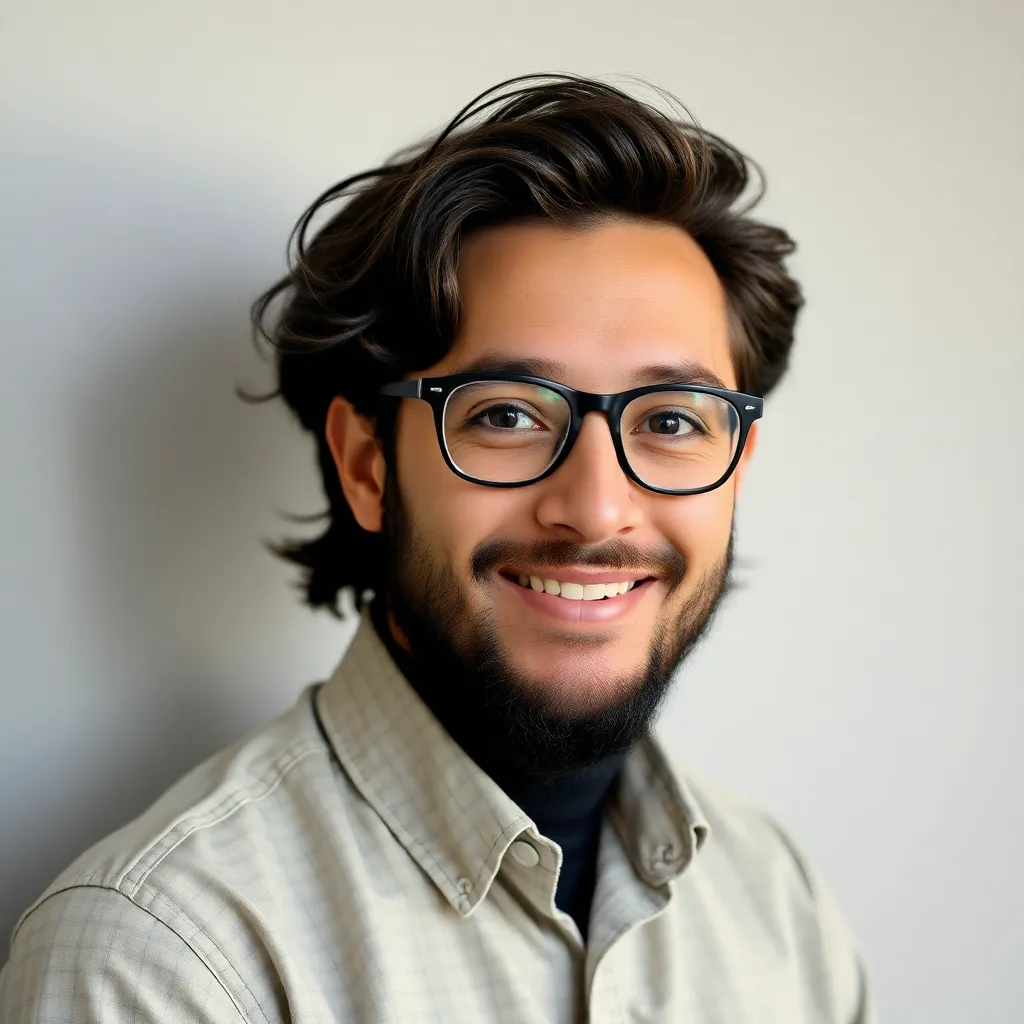
New Snow
Apr 27, 2025 · 7 min read

Table of Contents
As the Sample Size Increases: Exploring the Power of Larger Datasets in Statistical Analysis
Understanding the relationship between sample size and statistical analysis is crucial for researchers and data analysts alike. The core principle is simple: as the sample size increases, the accuracy and reliability of statistical inferences improve. This seemingly straightforward statement underpins a wealth of statistical theory and practice, impacting everything from experimental design to the interpretation of survey results. This article delves deep into this fundamental concept, exploring its implications and practical applications across various statistical methods.
The Central Limit Theorem and the Power of Numbers
At the heart of the sample size's impact lies the Central Limit Theorem (CLT). This cornerstone of statistics states that the distribution of the sample means from a large number of independent, identically distributed random variables will approximate a normal distribution, regardless of the original population's distribution (provided certain conditions are met). This is incredibly powerful because it allows us to make inferences about the population mean even if we don't know the true population distribution.
Smaller Samples: Higher Variability, Lower Precision
With small sample sizes, the sampling distribution of the mean is often highly variable. This means that the sample mean might deviate significantly from the true population mean, leading to unreliable estimates. Confidence intervals, which provide a range of values likely to contain the true population parameter, will be wider, reflecting this increased uncertainty. Hypothesis tests, designed to assess whether there's enough evidence to reject a null hypothesis, will have lower power—meaning there's a greater chance of failing to detect a true effect (Type II error).
Imagine trying to estimate the average height of adult women based on a sample of only five individuals. It's highly likely that the sample mean will be quite far from the true population average due to the inherent variability within the sample. This highlights the inherent limitation of relying on small datasets.
Larger Samples: Reduced Variability, Increased Precision
As the sample size increases, the variability of the sampling distribution decreases. This means that the sample mean is more likely to be closer to the true population mean. Consequently, confidence intervals become narrower, offering a more precise estimate of the population parameter. Hypothesis tests gain power, making it more likely that a true effect will be detected. This enhanced precision and power are the key benefits of using larger samples.
In our height example, if we increase the sample size to, say, 500 women, the sample mean will be a much more reliable estimate of the true average height. The confidence interval will be much narrower, reflecting the reduced uncertainty. Hypothesis tests investigating the difference in average height between two groups will have a higher chance of detecting a statistically significant difference, if one exists.
Impact on Different Statistical Methods
The influence of sample size extends across various statistical methods:
1. Confidence Intervals: Narrowing the Uncertainty
Confidence intervals provide a range of values within which we expect the true population parameter to lie with a certain level of confidence (e.g., 95%). As the sample size increases, the width of the confidence interval decreases, reflecting a more precise estimate of the population parameter. This is because larger samples lead to a smaller standard error, which is directly related to the width of the confidence interval.
2. Hypothesis Testing: Increasing Power
Hypothesis testing aims to determine whether there is sufficient evidence to reject a null hypothesis. The power of a hypothesis test, the probability of correctly rejecting a false null hypothesis, increases with sample size. Larger samples lead to smaller standard errors, making it easier to detect statistically significant differences or effects, even if they are small in magnitude. This reduces the risk of a Type II error (failing to reject a false null hypothesis).
3. Regression Analysis: Improving Model Accuracy
In regression analysis, larger sample sizes are crucial for obtaining stable and reliable estimates of regression coefficients. Larger samples reduce the impact of outliers and improve the precision of the estimates. They also allow for the inclusion of more predictor variables without overfitting the model, leading to more accurate predictions. This leads to more robust and generalizable models.
4. ANOVA (Analysis of Variance): Enhanced Sensitivity
ANOVA is used to compare the means of multiple groups. Similar to hypothesis testing, increasing the sample size in ANOVA increases the power of the test to detect differences between group means, even if those differences are small. This increased sensitivity is crucial for identifying subtle effects that might be missed with smaller samples.
5. Non-parametric Tests: Still Benefit from Larger Samples
While non-parametric tests don't rely on assumptions about the distribution of the data, they still benefit from larger sample sizes. Larger samples provide more information about the population, leading to more precise estimates and more powerful tests. Even though the assumptions are relaxed, the underlying principle of reducing variability through larger samples still applies.
Practical Considerations and Challenges
While larger sample sizes generally lead to more accurate and reliable results, there are practical considerations:
1. Cost and Feasibility: The Trade-off Between Resources and Precision
Collecting larger samples often incurs higher costs in terms of time, resources, and money. Researchers need to balance the desire for greater precision with the practical limitations of data collection. A cost-benefit analysis might be necessary to determine the optimal sample size, balancing the improved accuracy against the increased cost.
2. Data Quality: More Data Doesn't Always Mean Better Data
It's crucial to remember that more data doesn't automatically translate to better data. Large sample sizes can amplify the effects of biases or errors present in the data collection process. It's essential to ensure the data is accurate, reliable, and representative of the population of interest. Poor quality data, even in large quantities, can lead to misleading conclusions.
3. Data Management and Analysis: The Computational Challenges
Managing and analyzing large datasets can be computationally intensive. Researchers need appropriate computational resources and expertise to handle the data effectively. Advanced statistical software and techniques might be required to process and analyze large datasets efficiently.
Determining the Appropriate Sample Size: A Crucial Step
Choosing the appropriate sample size is crucial for ensuring the validity and reliability of statistical inferences. Several factors influence the determination of sample size, including:
- Desired level of precision: A higher level of precision (narrower confidence intervals) requires a larger sample size.
- Desired power of the test: Higher power (reduced risk of Type II error) necessitates a larger sample size.
- Variability in the population: Higher population variability requires a larger sample size to achieve the same level of precision.
- Significance level (alpha): A lower significance level (e.g., 0.01 instead of 0.05) generally requires a larger sample size.
- Number of groups or variables: More groups or variables often necessitate larger sample sizes.
There are various methods for calculating the required sample size, including power analysis techniques. These methods consider the factors mentioned above to determine the minimum sample size needed to achieve the desired level of precision and power.
Conclusion: Embracing the Power of Larger Samples
The relationship between sample size and the accuracy and reliability of statistical inferences is undeniable. As the sample size increases, the precision of estimates improves, confidence intervals narrow, hypothesis tests gain power, and the overall reliability of statistical conclusions strengthens. While practical limitations regarding cost and data management exist, the benefits of larger sample sizes in improving the quality of research and data analysis are significant. Researchers and data analysts should strive to collect sufficiently large samples to ensure the validity and reliability of their findings, acknowledging that this often necessitates careful planning, resource allocation, and a commitment to data quality. By understanding the fundamental principles discussed in this article, researchers can significantly enhance the credibility and impact of their work.
Latest Posts
Latest Posts
-
The Primary Goal Of A Hospital Based Cancer Registry Is To
Apr 28, 2025
-
Que Es El Espiritu De Jezabel
Apr 28, 2025
-
The Icd 10 Pcs Code For Tracheoscopy Is
Apr 28, 2025
-
A Counselor Who Is Beginning A Group Should
Apr 28, 2025
-
Where Does The Citric Acid Cycle Occur In Eukaryotes
Apr 28, 2025
Related Post
Thank you for visiting our website which covers about As The Sample Size Increases The . We hope the information provided has been useful to you. Feel free to contact us if you have any questions or need further assistance. See you next time and don't miss to bookmark.