Agrarian Technology Developed Based On The Use Of
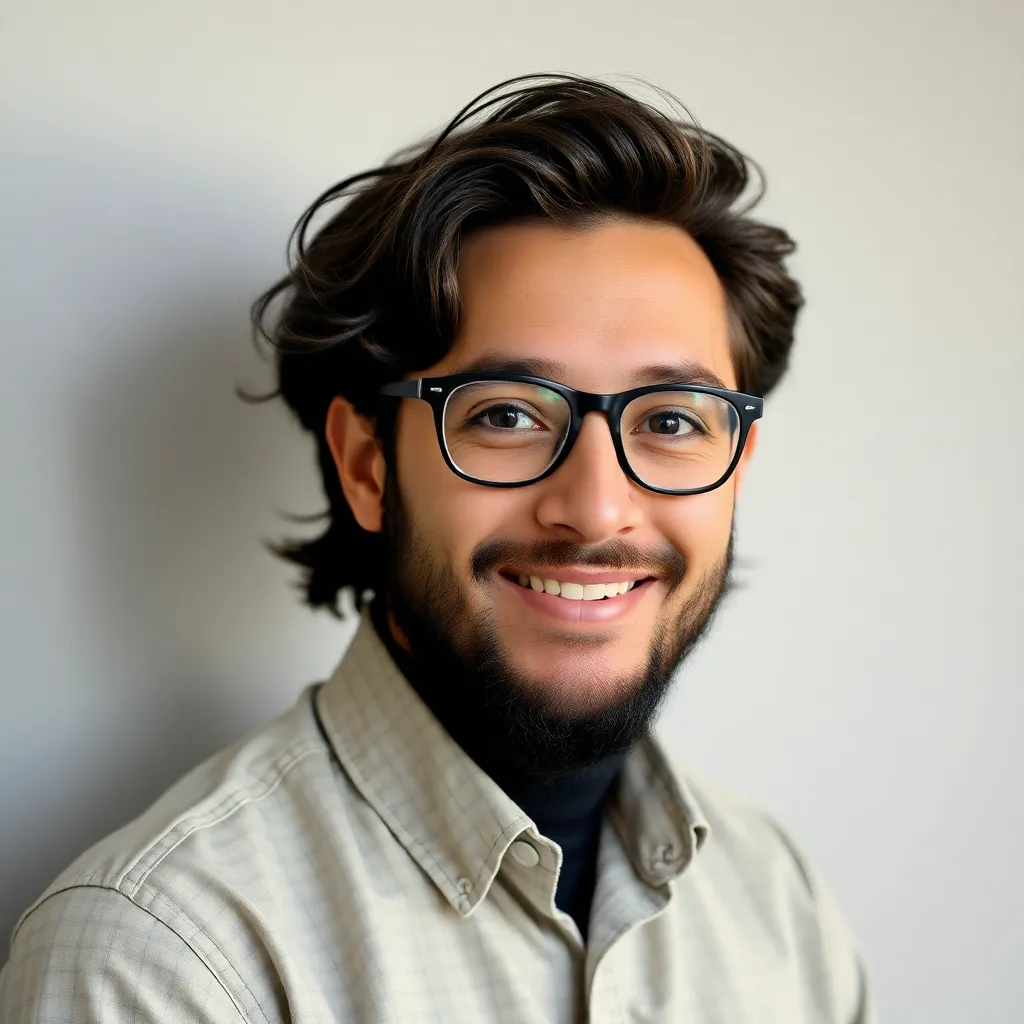
New Snow
Apr 25, 2025 · 6 min read

Table of Contents
Agrarian Technology Developed Based on the Use of AI and Machine Learning
The agricultural sector is undergoing a significant transformation, driven by the rapid advancements in artificial intelligence (AI) and machine learning (ML). These technologies are no longer futuristic concepts; they are actively shaping modern farming practices, boosting efficiency, and ensuring sustainable food production. This article delves into the diverse applications of AI and ML in agrarian technology, exploring their impact on various aspects of agriculture, from precision farming to crop monitoring and beyond.
Precision Farming: Optimizing Resource Utilization
Precision farming, a cornerstone of modern agriculture, leverages AI and ML to optimize resource allocation and minimize waste. This approach moves away from the traditional "one-size-fits-all" methods towards a highly targeted approach tailored to the specific needs of each field.
Variable Rate Technology (VRT): Tailoring Inputs for Maximum Yield
AI-powered VRT systems analyze data from various sources, including soil sensors, satellite imagery, and drone surveys, to create detailed maps of field variability. These maps identify areas requiring different levels of fertilizer, pesticides, water, and seeding. By applying these inputs precisely where needed, VRT significantly reduces waste, minimizes environmental impact, and maximizes crop yields. This data-driven approach contributes to substantial cost savings for farmers and reduces the overuse of potentially harmful chemicals.
Smart Irrigation: Conserving Water Resources
Water scarcity is a growing concern globally. AI-powered irrigation systems utilize sensors and weather forecasts to determine the precise water needs of crops, optimizing irrigation schedules and minimizing water waste. Machine learning algorithms can analyze historical data and predict future water requirements, enabling proactive water management and preventing water stress in crops. This intelligent approach to irrigation is crucial for sustainable agriculture, particularly in arid and semi-arid regions.
Crop Monitoring and Disease Detection: Early Intervention for Enhanced Productivity
Early detection of crop diseases and pests is critical for preventing widespread damage and minimizing yield losses. AI and ML are revolutionizing disease and pest detection through image analysis and predictive modeling.
Image Recognition and Analysis: Identifying Threats Quickly and Accurately
AI-powered image recognition systems can analyze images captured by drones, satellites, or even handheld devices to identify signs of disease, pest infestations, or nutrient deficiencies. These systems can detect subtle visual cues that might be missed by the human eye, allowing for timely interventions and preventing significant yield losses. The speed and accuracy of AI-based disease detection significantly enhance the effectiveness of pest and disease management programs.
Predictive Modeling: Forecasting Disease Outbreaks
ML algorithms can analyze historical data on weather patterns, disease incidence, and crop conditions to predict future disease outbreaks. This predictive capability empowers farmers to proactively implement preventative measures, such as adjusting planting schedules or applying appropriate treatments, before outbreaks occur. This proactive approach is crucial for mitigating the impact of diseases and ensuring the health of crops.
Robotics and Automation: Enhancing Efficiency and Reducing Labor Costs
The integration of robotics and automation in agriculture is streamlining various farming operations, reducing labor costs, and increasing efficiency.
Autonomous Tractors and Harvesting Machines: Optimizing Farm Operations
Self-driving tractors and harvesting machines guided by GPS and AI are transforming field operations. These autonomous machines can operate around the clock, optimizing efficiency and reducing labor requirements. They are particularly valuable in addressing labor shortages and enhancing the speed and precision of tasks such as planting, spraying, and harvesting.
Robotic Weeding: Precision Weed Control
Robotic weeders use computer vision and AI to identify and remove weeds with precision, minimizing the need for herbicides. This targeted approach to weed control reduces chemical usage, protects beneficial insects, and enhances environmental sustainability. Robotic weeding is a significant step towards sustainable and environmentally friendly agricultural practices.
Supply Chain Optimization: Enhancing Logistics and Reducing Waste
AI and ML are not only impacting on-farm operations but also transforming the agricultural supply chain.
Predictive Analytics: Optimizing Logistics and Reducing Spoilage
Predictive analytics can analyze data from various sources, including weather forecasts, market demand, and transportation routes, to optimize logistics and minimize spoilage. By predicting potential disruptions and optimizing transportation routes, AI helps ensure that produce reaches its destination in optimal condition, reducing waste and maximizing profitability.
Inventory Management: Preventing Shortages and Waste
AI-powered inventory management systems provide real-time insights into supply and demand, helping farmers and distributors make informed decisions about production and distribution. This prevents shortages, reduces waste, and optimizes the utilization of resources throughout the supply chain. Efficient inventory management is critical for ensuring food security and meeting consumer demand.
Data Analytics and Decision Support Systems: Empowering Informed Decision-Making
The vast amounts of data generated by modern agricultural technologies require sophisticated analytical tools to extract meaningful insights. AI and ML provide the necessary capabilities to process this data and empower informed decision-making.
Data Integration and Analysis: Gaining Comprehensive Insights
AI-powered platforms can integrate data from various sources, including sensors, GPS devices, weather stations, and market data, to provide a comprehensive overview of farm operations. This integrated data analysis provides farmers with actionable insights to optimize their practices and make informed decisions about resource allocation, pest management, and crop planning.
Decision Support Systems: Guiding Farmers Towards Optimal Strategies
AI-powered decision support systems use machine learning algorithms to analyze data and provide recommendations to farmers. These systems can guide farmers on optimal planting times, fertilizer application rates, irrigation schedules, and pest management strategies, maximizing yields and minimizing risks. Decision support systems empower farmers to make data-driven decisions, improving efficiency and profitability.
The Future of AI and ML in Agrarian Technology: Ongoing Innovations
The integration of AI and ML in agriculture is an ongoing process, with continuous innovation driving further advancements.
Enhanced Sensor Technologies: Gathering More Precise Data
Advances in sensor technology will enhance the accuracy and detail of data collected from fields. New sensors will provide more precise information on soil conditions, crop health, and environmental factors, leading to more accurate predictions and optimized farming practices.
Improved Algorithms and Models: Refining Predictive Accuracy
Ongoing research and development in machine learning will lead to more accurate and sophisticated predictive models. These improved algorithms will enable more precise predictions of crop yields, disease outbreaks, and other factors, further enhancing the effectiveness of AI-powered agricultural technologies.
Increased Accessibility and Affordability: Democratizing Technology
As AI and ML technologies become more accessible and affordable, their adoption will expand across a wider range of farms, particularly smallholder farms. This democratization of technology will empower farmers of all sizes to leverage the power of AI and ML to improve their productivity and sustainability.
Conclusion: A Sustainable and Efficient Future for Agriculture
The integration of AI and machine learning into agrarian technology is transforming the agricultural sector, paving the way for a more sustainable, efficient, and productive future. From precision farming to crop monitoring and supply chain optimization, these technologies are enhancing various aspects of agriculture, ensuring food security, and promoting environmental sustainability. As AI and ML technologies continue to evolve, their impact on agriculture will only intensify, shaping the future of food production globally. The continuous advancements in this field offer immense potential for addressing global challenges related to food security, resource management, and environmental protection, ultimately leading to a more resilient and sustainable agricultural system for generations to come.
Latest Posts
Latest Posts
-
Which Of The Following Cells Transport Sugars Over Long Distances
Apr 25, 2025
-
Which Of The Following Multiple Intelligences Best Describes An Athlete
Apr 25, 2025
-
A Nurse Is Administering Aspirin 81 Mg
Apr 25, 2025
-
What Is The Formula For The Ionic Compound Ammonium Nitrate
Apr 25, 2025
-
Which Is A Characteristic Of Colloids
Apr 25, 2025
Related Post
Thank you for visiting our website which covers about Agrarian Technology Developed Based On The Use Of . We hope the information provided has been useful to you. Feel free to contact us if you have any questions or need further assistance. See you next time and don't miss to bookmark.